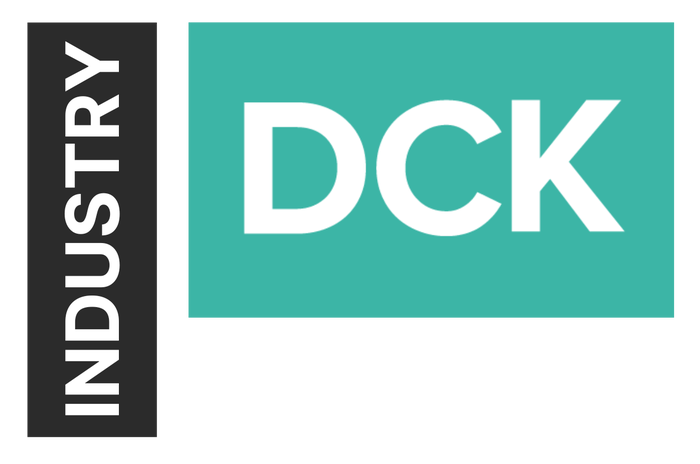
Insight and analysis on the data center space from industry thought leaders.
Evaluating Predictive Analytics for Flash Arrays
The right predictive analytics system should be able to automate level 1 and 2 support and troubleshooting entirely.
March 7, 2017
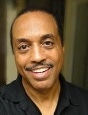
Andre Franklin is Senior Product Marketing Manager for Nimble Storage.
Flash storage is rapidly becoming the norm in the enterprise data center. Since flash inherently delivers high speed, buyers are starting to evaluate vendors on criteria other than speed and table stakes features. Predictive analytics is among the capabilities being regularly evaluated as it promises far more than raw storage speed. That said, predictive analytics is relatively new in the long history of storage, which makes it difficult to effectively evaluate the features and benefits of any given vendor’s predictive analytics capabilities.
What makes the challenge even more difficult is that predictive analytics doesn’t yet have a widely agreed upon definition. And when vendors themselves disagree on what a technology is, it’s not a surprise that customers likewise won’t know how to evaluate it.
So, for the moment, in the storage context, let’s agree on three things that predictive analytics is NOT:
A re-labeling or re-naming of age-old common storage capabilities, such as capacity reporting and projections, reporting from hard fault sensors, or analysis of log files
A reactive system that responds to issues after the fact
A tool that assumes most issues are rooted in the storage system itself (research across thousands of issues and thousands of users revealed that application slowdowns are blamed on storage most of the time, but that storage is actually at fault less than half the time)
Predictive Analytics and the Maytag Repairman
Years ago, Maytag televised an ad campaign with the focal point being a Maytag repairman. Maytag was well-known as a manufacturer of washing machines in those days. Unlike the image of a repairman always rushing from job-to-job, the Maytag man was, unintentionally, the least busy man in the neighborhood. Although not his fault, there was little work to do because the Maytag washing machines never broke down.
Clearly, the goal of all IT departments is perfect reliability and totally effortless management. Put another way, modern IT departments aspire to have “Maytag” systems so that nothing ever goes wrong. Of course, IT resources are not going to sit by the phone (or their workstation) all day waiting for trouble tickets that never come in. Instead, IT can reassign them to more strategic tasks.
Realistically, the complexities of the modern data center make the Maytag dream impossible without something to address complexity unlike anything the Maytag repairman ever faced.
That “something” is predictive analytics.
What Is Predictive Analytics for Infrastructure?
True predictive analytics eliminates the stress of managing infrastructure. It uses machine learning and data science to predict, diagnose, and prevent problems, using data collected from the infrastructure stacks across a large base of customers. This keeps IT infrastructure running optimally, while also enabling IT to anticipate future needs.
Customers evaluating the predictive analytics capabilities of flash storage products should understand and evaluate the following capabilities:
Ability to proactively predict and prevent problems
Global visibility so that data and analytics can take the complete environment into account and correlate it with data collected from all other environments
An analytics-based support experience that is vastly beyond what would have been possible otherwise
Predict and Prevent
If you can predict, you should be able to prevent. The two should go hand-in-hand for IT infrastructure. But in order to reach this level of functionality, IT infrastructure should get smarter over time. Once the system encounters a complicated problem, it should learn from the experience so the same issue doesn’t arise again.
A vendor may state that their flash storage environment can calculate when a disk drive will reach capacity, based on how much a customer is utilizing per week. But calculating the duration of time before it reaches capacity is easy. Yes, technically it’s predictive. But what’s the true benefit? These types of capabilities are rudimentary, and have existed for more than 30 years.
The ultimate goal is to achieve a truly self-healing infrastructure. Whether compute, network or storage, the problem should be identified, and often times resolved, with no human intervention required and before the business is adversely affected.
Global Visibility
A common limitation in predictive analytics capabilities is the inability to look across the entire environment and across other customer environments when analyzing an issue. In today’s complex data center infrastructures, that’s a crippling limitation, because IT is left with the typical, frustrating circle of finger pointing: whoever made the network switch blames storage, the storage vendor blames the network, and the cycle goes on, ad infinitum.
Take, for instance, a storage solution that only looks at itself. As mentioned above, recent research illustrates that 46 percent of performance related issues are in the storage environment. But what about the other 54 percent? Configuration errors, interoperability and poor IT practices are among the many other culprits.
Instead of trying to track down the offender, it’s much easier to be handed the diagnoses for the problem with actionable insights, or even better, automated adjustments. When predictive analytics examine granular data across the infrastructure stack – network, storage and compute – the pain of firefighting and troubleshooting is dramatically reduced. This becomes even more powerful when a particular environment can be compared against other environments to determine if it is operating correctly. As a result, IT can focus on more strategic directives that drive business success.
A Transformed Support Experience
When predictive analytics are utilized appropriately, the result is a support experience unlike any other.
Customer satisfaction hinges on the support experience. That’s why it’s imperative for businesses to get out in front of it. For years, the model has been that the customer calls the company when a problem persists. Technological advances have come too far, too fast for this model to be considered suitable. Companies should call customers to pre-empt problems; not the other way around.
IT shouldn’t have to spend countless hours troubleshooting, and businesses shouldn’t have to waste time talking to level 1 and level 2 customer support before finally being sent to level 3. Companies can’t afford to go days without an answer. Yet, many vendors apparently believe this is the way life is supposed to be. The right predictive analytics system should be able to automate level 1 and 2 support and troubleshooting entirely.
There are plenty of “me too” claims coming out of the flash market. But in many cases, these predictive capabilities are very limited in functionality and add little value to the customer.
The data center should be experiencing the return of the Maytag spirit. Predictive analytics done right prompts enhanced performance and reliability, and ultimately a better customer experience, from firefighting to customer support engagements. In short, these capabilities should act like a built-in IT admin of sorts, or better yet, IT’s own Maytag repair man.
Opinions expressed in the article above do not necessarily reflect the opinions of Data Center Knowledge and Penton.
Industry Perspectives is a content channel at Data Center Knowledge highlighting thought leadership in the data center arena. See our guidelines and submission process for information on participating. View previously published Industry Perspectives in our Knowledge Library.
About the Author
You May Also Like