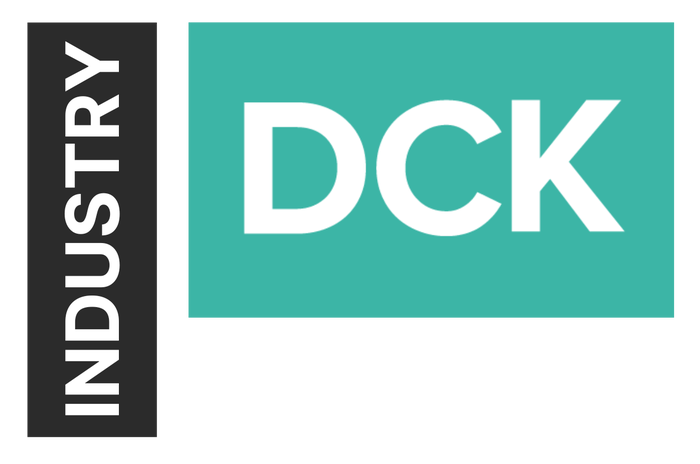
Insight and analysis on the data center space from industry thought leaders.
AI Ushers In the New Day-to-Day for Data Center Teams
AI has moved from far off imagination to short-term imperative. What will artificial intelligence mean for the data center industry? And how will people get started?
March 18, 2023
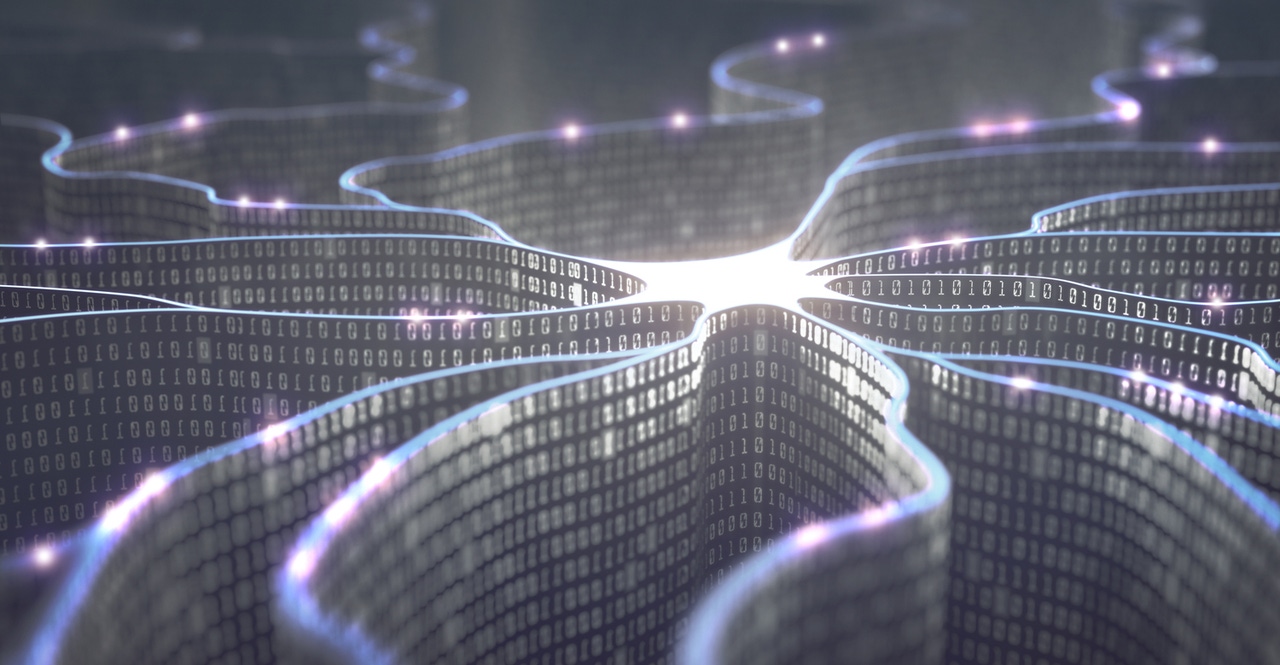
The buzziest of buzzwords in at least a decade has to be artificial intelligence (AI). Fueled in part by breakthrough use cases in generated text, art and video, AI has moved from far off imagination to short-term imperative. It’s impacting the way people think about all manners of fields, and data center networking is certainly not immune. But what will AI likely mean in the data center? And how will people get started?
Operations as the battleground
While it is possible that researchers might unlock some algorithmic approach to network control, that doesn’t seem the likely dominant use case for AI in the data center. The simple truth is that data center connectivity is largely a solved problem. Esoteric capabilities and micro-optimizations might yield tangible benefits over a hyperscale environment, but for the mass market, it’s probably unnecessary. If it were critical to do this, the move to cloud would be gated by the emergence of tailored networking solutions, but alas, that’s not the case.
If AI is going to make a lasting impression, it’s must be on the operations side. The practice of networking—the workflows and activities required to make networks happen—will be the battleground. Juxtaposed with the industry’s 15-year ambitions around automation, this actually makes good sense. Might AI provide the technological boost required to finally move the industry from dreaming about operational advantage to actively leveraging automated, semi-autonomous operations?
Deterministic or stochastic?
It seems possible, but there is nuance in the answer to this question. At a macro level, there are two different operational behaviors in data center: those that are deterministic and lead to known outcomes, and those that are stochastic or probabilistic.
For workflows that are deterministic, AI is not just overkill; it’s entirely unnecessary. More specifically, for a known architecture, the configuration required to drive the devices doesn’t require an AI engine to process. It requires a translation from architectural blueprint to device-specific syntax. Even in the most complex cases (multivendor architectures with varying scale requirements), the configuration can be entirely predetermined. There might be nested logic to handle variation in device type or vendor configuration, but nested logic hardly qualifies as AI.
But even beyond configuration, many of the Day 2 operational tasks don’t require AI. For example, take one of the more common use cases that marketers have branded with AI for years: resource thresholds. The logic is that AI can determine when key thresholds like CPU or memory utilization are crossed, and then take some remediating action. Thresholding is not really that complicated. The mathematicians and AI purists might comment that linear regression is not really intelligence. Rather, it’s fairly crude logic based on trend lines, and importantly, these things have been in various production environments since before AI was a fashionable term.
So, does that mean that there is no role for AI? Absolutely not! It does mean that AI isn’t a requirement or even a fit for everything, but there are workflows in networking that can and will benefit from AI. Those workflows that are probabilistic rather than deterministic will be the best candidates.
Troubleshooting as a potential candidate
There is perhaps no better candidate for probabilistic workflows than root cause analysis and troubleshooting. When something goes wrong, network operators and engineers go through a set of activities designed to rule things out and hopefully identify the root cause. For simple issues (think: things that someone might get resolved with a tier-1 trouble ticket), the workflows are probably scripted (“Have you tried rebooting the device?”). But for anything beyond the most basic problems, the operator is applying some logic and selecting the most likely but not predetermined path forward. Based on what the individual knows or has learned, there is some refinement, and either more information is sought or a guess is made.
AI has a role to play here. And we know this implicitly because we understand the value of experience in the troubleshooting process. A new employee, however skilled they might be, will typically underperform someone with a very long tenure who knows where all the proverbial bodies are buried. AI can act as a substitute for or supplement to all of that ingrained experience, and recent advances in natural language processing (NLP) help smooth the human-machine interface.
AI starts with data
The best wine starts with the best grapes. Similarly, the best AI will begin with the best data. This means that well-instrumented environments will prove to be the most fertile for AI-driven operations. The hyperscalers are certainly further along the AI path than others, due in large part to their software expertise. But it ought not be discounted that they build their data centers with a ton of emphasis on gathering information in real time through streaming telemetry and large-scale collection frameworks.
Companies that want to leverage AI at some point ought to be examining their current telemetry capabilities. Basically, does the existing architecture help or hinder any serious pursuits? And then architects need to be building these operational requirements into the underlying architectural evaluation process. Too often in enterprises, operations is an afterthought—some add-on that happens after the equipment has gone through the purchasing department. That cannot be the norm for any data center that aspires one day to leverage anything more than lightly scripted operations.
Going back to the question of deterministic or stochastic, the question really ought not be framed as an either-or proposition. There is a role to play for both. Every data center will feature a set of deterministic workflows, and there is opportunity to do some breakthrough things in the probabilistic world. Both of these will benefit from data. So, regardless of the ambitions and starting point, everyone ought to be focused on data.
Tempering expectations
The key to success for most enterprises will be tempering expectations. The future is sometimes defined by grand proclamations, but more often than not, the grander the vision, the more unattainable it seems. And when the to-be state is too far removed from the as-is state, companies and people shut down because it’s a chasm that is too wide to bridge.
What if the next wave of advancement was powered more by boring innovation than over-the-top promises? What if reducing trouble tickets and human error was compelling enough to get people started? Aiming at the right targets will make growth so much easier for people. This is especially true in an environment that feels starved for enough talented people to staff everyone’s ambitious agendas. So even as AI trends into the Trough of Disillusionment over the next couple of years, the opportunity for data center operators to make a meaningful difference for their businesses will still be there.
About the Author
You May Also Like