Top Three Use Cases for AI in Cybersecurity
Artificial intelligence systems can help detect zero-day malware, prioritize threats, and take automated remediation actions.
February 3, 2022
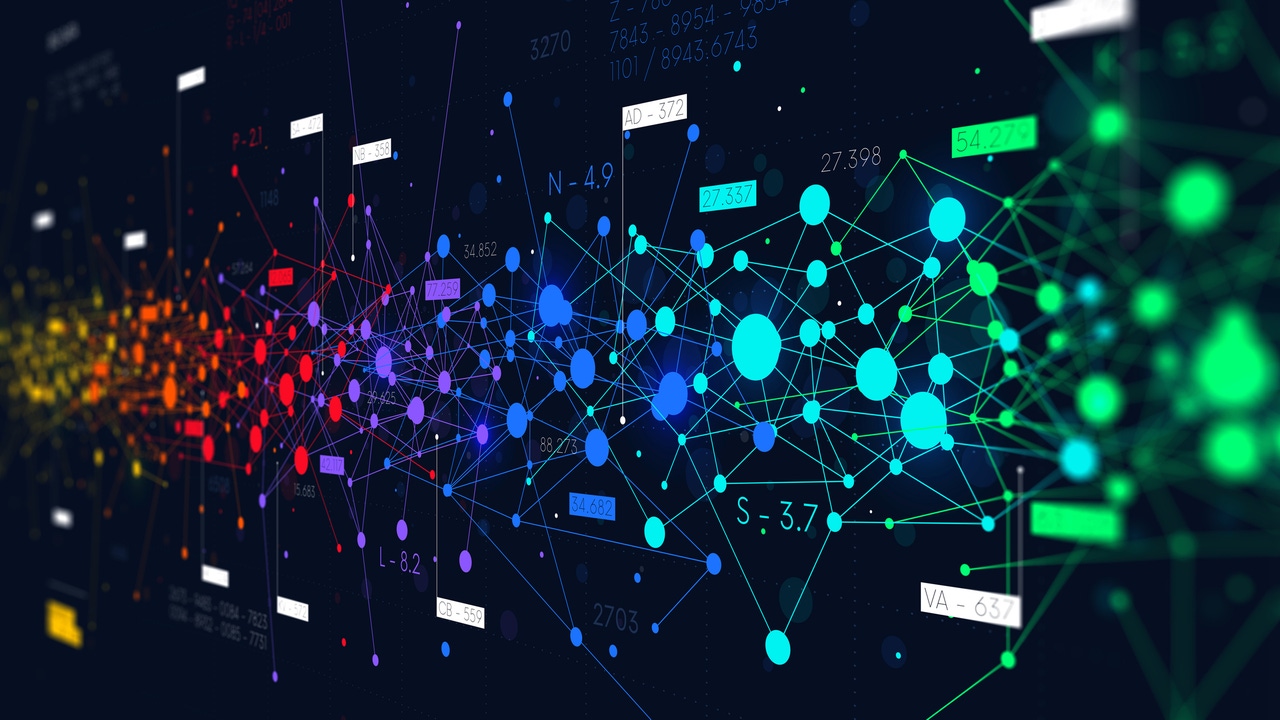
Cybersecurity professionals are facing an unprecedented threat environment, with record-high numbers of attacks, shortage of qualified staff, and increasing aggression and sophistication from nation-state actors.
For many data center cybersecurity managers, the silver bullet for all these problems is artificial intelligence. It promises to allow security teams to handle more threats than ever before, of greater complexity than ever before, with fewer and fewer people.
In fact, according to a global survey released this past September by Pillsbury, a global law firm focusing on technology, 49% of executives think artificial intelligence is the best tool to counter nation-state cyber attacks.
And they’re putting their money on it. Pillsbury predicts that cybersecurity-related AI spending will increase at a compound annual growth rate of 24% through 2027 to reach a market value of $46 billion.
The use of machine learning is widespread across cybersecurity, said Omdia analyst Fernando Montenegro. Its applications include classification algorithms used for malware and spam detection, anomaly detection algorithms used to detect malicious traffic or user behaviors, and correlation algorithms used to connect signals from disparate systems.
“Usually any cybersecurity tool or product that is implementing these use cases will likely be using machine learning techniques,” he told Data Center Knowledge.
More specifically, experts say, artificial intelligence and machine learning are already proving their worth in spotting zero-day malware, identifying and prioritizing threats, and, in some cases, taking automated actions to quickly remediate security issues at scale.
Zero-day malware
Attackers are getting extremely efficient at creating updated versions of malware, ones that can evade signature-based detection.
Last year, the AV-Test Institute logged more than 1.3 billion new pieces of malware and potentially unwanted applications.
According to a July report by Ernst & Young, 77% of global executives saw an increase in disruptive attacks such as ransomware over the past year, compared to 59% the year before.
AI and ML-powered systems can analyze malware based on inherent characteristics, rather than signatures. For example, if a piece of software is designed to rapidly encrypt many files at once, that’s a suspicious behavior. If it takes steps to hide itself from observation, that’s another sign that the software isn’t legitimate.
An AI-based tool can look at these characteristics, and many others, in order to calculate the risk of a new, previously-unseen piece of software.
“AI can tag things as malware that don’t look like prior malware samples,” said Kayne McGladrey, IEEE senior member and cybersecurity strategist at Ascent Solutions.
The result can be a dramatic improvement in endpoint security.
Legacy, signature-based technology is effective at stopping 30% to 60% of threats, said Chuck Everette, director of cybersecurity advocacy at Deep Instinct. “Machine learning takes the effectiveness up to 80% to 92%.”
With more employees working from home since the start of the pandemic, endpoint security has become much more critical.
Ransomware reached a record high last year, reported Expel, the managed detection and response provider, in a report released last week.
And eight out of ten ransomware attacks were self-installed — users unwittingly infected their networks by opening a malicious file containing malware.
“Endpoint security is an excellent case study,” said Steve Carter, co-founder and CEO at Nucleus Security. “Nearly every vendor in that space has developed and trained machine-learning systems to identify anomalous system and user behavior in real time to block both known and unknown malware from executing.”
Previous systems used a list of known signatures of bad programs, he told Data Center Knowledge. “The modern way is to try and detect previously unknown malicious programs.”
Identifying and prioritizing threats
Security operations center analysts are overwhelmed by security alerts that come in every day, many of them false positives. They wind up spending too much of their time doing routine work, and not enough time working on the big problems — or miss those advanced attacks altogether.
“All vendors have to use AI and ML today, just to handle the volume of threats and the sophistication of threats,” said Etay Maor, cybersecurity professor at Boston College and senior director of security strategy at Cato.
In a Trend Micro survey of IT security and SOC decision-makers released last May, 51% said they were overwhelmed by the volume of alerts and 55% said they weren’t confident in their ability to prioritize and respond to them.
In addition, according to the survey, respondents spent up to 27% of their time dealing with false positives.
Meanwhile, actual positives can easily be missed.
According to a survey of SOC professionals by Critical Start released in March, nearly half turn off high-volume alerting features when there are too many alerts to process.
There were over 900 attacks per organization per week in the fourth quarter of last year, an all-time high, according to a Check Point report released last month.
The overall number of attacks on corporate networks was up 50% last year, compared to 2020.
According to Verizon’s data breach investigation report, 20% of breaches took months or longer before organizations realized something was amiss.
Correlating disparate events inside a corporate environment and figuring out which ones indicate an actual threat is something that artificial intelligence can do well.
“The big things we’re seeing effectively in cybersecurity right now around AI is security incident and event management,” said Ascent Solutions’ Kayne McGladrey.
The reason is that it involves large pattern analysis, McGladrey told Data Center Knowledge, and AI is very good at doing large pattern analysis.
“It does that at a scale and speed that human defenders cannot match,” he said.
Taking automated actions
Finally, artificial intelligence and machine learning can be used to automate repetitive tasks, such as responding to the high volumes of low-risk alerts.
These are alerts where a response needs to be fast but the risks of making a mistake are low and the system has a high degree of certainty about the threat. For example, if a known sample of ransomware shows up on an end user’s device, immediately shutting down its network connectivity can save the rest of the company from a dangerous infection.
Intelligent automation can step in and take care of these problems when appropriate, helping companies deal with a shortage of qualified cybersecurity professionals.
Similarly, intelligent automation can be used to gather research about security incidents, pulling in data from multiple systems and assembling it into a report ready for analyst review, saving them a lot of routine effort.
“Ten years ago, people were afraid AI was going to take your job,” said Cato’s Maor. “It’s not going to take your job. It’s just going to take the noise away.”
Smart cybersecurity people are expensive and they’re hard to keep, he said.
“Let them do the smart stuff, the deep-dive investigations instead of trying to correlate different systems,” he said.
There’s a global shortage of 2.72 million cybersecurity professionals, according to the 2021 (ISC)2 cybersecurity workforce study released past October.
This is down from 3.12 million in 2020, due to 700,000 new entrants entering the field. But even with the influx of new talent, demand continues to greatly outpace supply. According to (ISC)2, the global cybersecurity workforce needs to grow 65% to effectively defend organizations’ critical assets.
The limits of AI
Artificial intelligence and machine learning show a great deal of promise, but it’s not a universal cure-all.
“While AI and ML technology are in some cases being leveraged to drive improvements in detection and response, organizations need to understand that artificial intelligence and machine learning are not answers in and of themselves,” said Joe McMann, global cybersecurity portfolio lead at Capgemini.
Despite its benefits, AI is not perfectly adapted to detect cyber threats, he said. For one, it doesn’t deal well with change, such as a sudden and unexpected pandemic completely shifting employees’ work behavior.
“Just like any tool or platform, in order to get the most return they must be integrated into the overall ecosystem, constantly tweaked and tuned, and measured for continued effectiveness,” McMann said.
The best use cases for the most advanced algorithms are those that don’t evolve much over time, since labeling data and training models are time consuming processes.
In many cases, enterprise networks in particular change too quickly for anomaly detection algorithms to be useful, said Nash Borges, VP of engineering and data science at Secureworks.
“The belief that anomaly detection can be used to learn about an enterprise environment’s normal behavior in order to generate meaningful alerts as soon as something abnormal occurs is more fantasy than reality,” he told Data Center Knowledge. “The digital behaviors of enterprise environments can’t really be baselined. They are fully dynamic systems, constantly responding to a spectrum of internal and external conditions.”
Anomalies are common, he said, and are rarely caused by malicious threat actors.
Another problem with using AI for cybersecurity incidents is that there’s a data imbalance in this sector that is greater than in many other fields.
A company might see 300 billion events a day, out of which less than a dozen are truly malicious incidents that could have severe consequences.
“So even if you had an amazing detector that was 99.999% accurate, you would be searching for those dozen true positives in a sea of 3 million false positives every day,” he said.
As a result of these and other limitations, while many vendors claim to have AI powered solutions, the technology might not yet be ready for prime-time — and many companies would be better off investing their resources into the fundamentals.
“There is a disproportionate fascination with chasing the shiny AI tools instead of focusing on getting the basic tenets of cybersecurity done correctly,” said Nucleus Security’s Carter.
Freelance cybersecurity journalist Alex Korolov contributed to this report.
About the Author
You May Also Like