What’s the Best Computing Infrastructure for AI?What’s the Best Computing Infrastructure for AI?
Charlie Boyle, the man in charge of Nvidia’s AI supercomputer line, on AI hardware and data centers today and in the future.
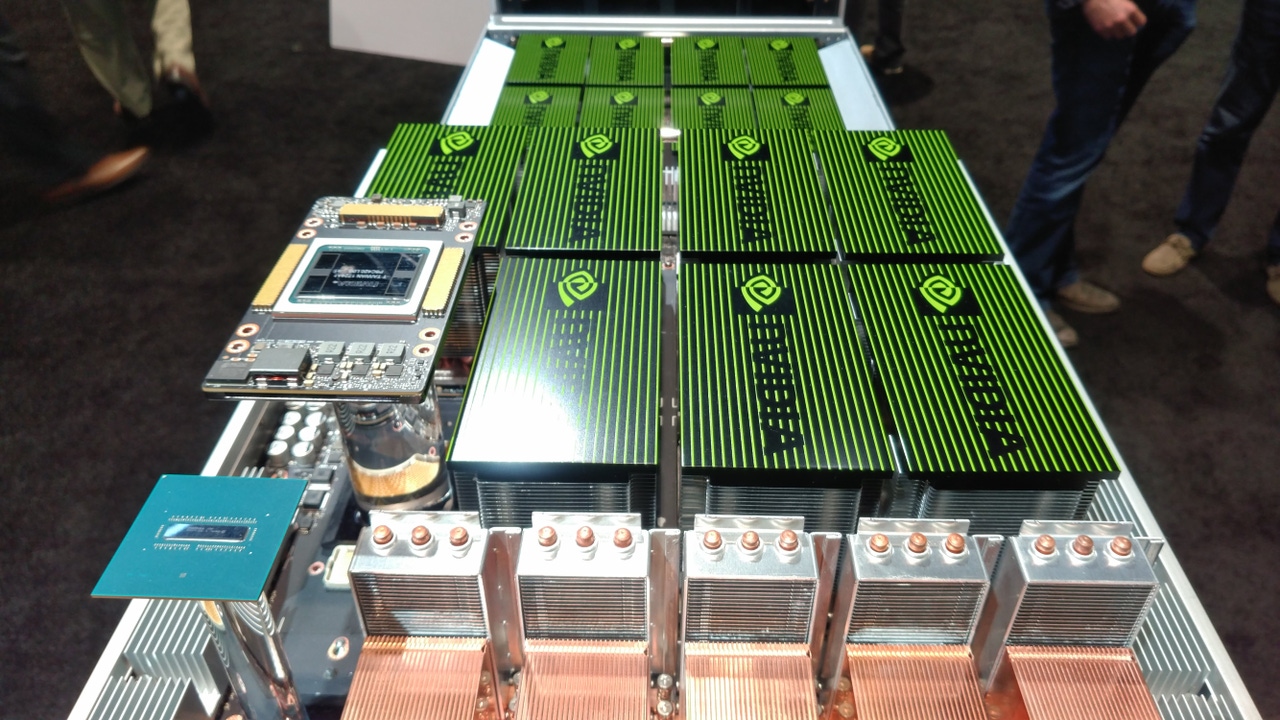
More than ever before, the most consequential question an IT organization must answer about every new data center workload is where to run it. The newest enterprise computing workloads today are variants of machine learning, or AI, be it deep learning-model training or inference (putting the trained model to use), and there are already so many options for AI infrastructure that finding the best one is hardly straight-forward for an enterprise.
There’s a variety of AI hardware options on the market, a wide and quickly growing range of AI cloud services, and various data center options for hosting AI hardware. One company that’s in the thick of it across this entire machine learning infrastructure ecosystem is Nvidia, which not only makes and sells most processors for the world’s AI workloads (the Nvidia GPUs), it also builds a lot of the software that runs on those chips, sells its own AI supercomputers, and, more recently, prescreens data center providers to help customers find ones that are able to host their AI machines.
We recently sat down with Charlie Boyle, senior director of marketing for Nvidia's DGX AI supercomputer product line, to talk about the latest trends in AI hardware and the broader world of AI infrastructure. Here’s our interview, edited for clarity and brevity:
DCK: How do companies decide whether to use cloud services for their machine learning workloads or buy their own AI hardware?
Charlie Boyle: Most of our customers use a combination of on-prem and the cloud. And the biggest dynamic that we see is location of the data influences where you process it. In an AI context, you need a massive amount of data to get a result. If all that data is already in your enterprise data center (you’ve got you know 10, 20, 30 years of historical data), you want to move the processing as close to that as possible. So that favors on-premises systems. If you’re a company that started out in the cloud, and all your customer data is in the cloud, then it’s probably best for you to process that data in the cloud.
Charlie Boyle, senior director of marketing for Nvidia's DGX AI supercomputer product line
And that’s because it’s hard to move a lot of data in and out of clouds?
It is, but it also depends on how you’re generating your data. Most customers’ data is pretty dynamic, so they’re always adding to it, and so, if they’re collecting all that data on premises in systems, then it’s easier for them to continue to process it on premises. If they’re aggregating lots of data into cloud services, then they process it in cloud services.
And that’s really for production use cases. Lots of experimental use cases may start in the cloud – you just fire up a browser and you get access to AI infrastructure – but as they move to production, customers make the locality decisions, the financial decisions, the security decisions, whether it’s better for them to process it on premises or in the cloud.
Nvidia customers typically do some AI model training on premises, because that’s where their historical data is. They build a great model, but the model is then served by their online services – the accelerated inference they do in the cloud based on the model they built on-premises.
For those running AI workloads on their own hardware on premises or in colocation data centers, what sort of cooling approaches have you seen, given how power-dense these boxes are?
The liquid-versus-air-versus-mixed-cooling is always an active debate, one that we do research on all the time. Generally, where we are today – people running handfuls of systems, maybe up to 50 or so – just conventional air cooling works fine. When we start getting into our higher-density racks, you know 30, 40, 50-kilowatt racks, we’re generally seeing rear-door, water-cooled heat exchangers, especially active rear door. And that’s what we implement in our latest data centers, because that way you’re not changing the plumbing on the physical system itself.
Now, some of our OEM partners have built direct-to-chip water cooling based on our GPUs as well, not for DGX. Some customers that are building a brand-new data enter and want to build a super dense supercomputing infrastructure, they’ll put that infrastructure in ahead of time.
But where we are with most colocation providers – and even most modern data centers people are building this year and maybe next year – it’s chilled water or ambient water temperature just to the rack level to support the rear door.
Direct-to-chip is more of an industry and operations issue. The technology is there, we can do it today, but then how do you service it? That’s going to be a learning curve for your normal operations people.
Do you DGX systems and other GPU-powered AI hardware will be so dense that you won’t be able to cool them with air, period?
All the systems I’m looking at in the relatively near future can either be air-cooled, water-rear-heat-door, or air-water in the rack. Mainly because that’s where I see most enterprise customers are at. There’s nothing inherent in what we need to do in density that says we can’t do air or mixed-air for the foreseeable future, mainly because most people would be limited by how much physical power they can put in a rack.
Cooling fans of the Nvidia DGX-2 system on display at GTC 2018
Right now, we’re running 30-40kW racks. You could run 100kW racks, 200kW racks, but nobody has that density today. Could we get to the point where you need water cooling? Maybe, but it’s really about the most efficient option for each customer. We see customers doing hybrid approaches, where they’re recovering waste heat and other things like that. We continue to look at that, continue to work with people that are building things in those spaces to see if it makes sense.
Our workstation product, the DGX station, is internally water-cooled, so one of our products is already closed-loop water-cooled. But from a server side, that data center infrastructure, most people aren’t there yet.
Most enterprise data centers aren’t capable of cooling even 30kW and 40kW racks. Has that been a roadblock for DGX sales?
It really hasn’t. It’s been a conversation point, but that’s also why we announced the second phase of our DGX-ready program. If you’re just talking about putting a few systems in, any data center can support a few systems, but when you get into the 50-100 systems, then you’re looking at rearchitecting the data center or going to a colo provider that already has it.
That’s why we really try to take the friction out of the system, partnering with these colo providers, having our data center team do the due diligence with them, so that they already have the density, they already have the rear-door water cooling that’s needed, so that customer can just pick up the phone and say hey, I need room for 50 DGX-2s, and the data center provider already has that data, puts it in their calculator and says, OK, we can have that for you next week.
So, there was friction?
As we rolled these products out three years ago, as people were buying a few systems at a time, they were asking questions about doing this at scale, and some of our customers chose to build new infrastructure, while others looked to us for recommendations on a good-quality close colocation provider. We built the DGX-Ready data center program so that customers wouldn’t have to wait.
Even for customers who had great data center facilities, many times the business side would call up the data center and say, oh, I need four 30kW racks. The data center team would say, great, we can do that, but that’s six months; alternatively, we can go to one of our colo partners, and they can get it next week.
Do you see customers opting for colo even if they have they have their own data center space available?
Since AI is generally a new workload for most customers, they’re not trying to back-fit an existing infrastructure. They’re buying all new infrastructure for AI, so for them it doesn’t really matter if it’s in their data center or in a colocation provider that’s close to them – as long as it’s cost effective and they can get the work done quickly. And that’s a really big part of most people’s AI projects: they want to show success really fast.
Even at Nvidia, we use multiple data center providers right near our office (DCK: in Santa Clara, California), because we have office space, but we don’t have data center space. Luckily, being in Silicon Valley, there’s great providers all around us.
Nvidia is marketing DGX as a supercomputer for AI. Is its architecture different from supercomputers for traditional HPC workloads?
About five years ago people saw a very distinct difference between an HPC and an AI system, but if you look at the last Top500 list, a lot of those capabilities have merged. Previously, everyone thought of supercomputing as 64 bit, double precision, scientific code for weather, climate, and all those different things. And then AI workloads were largely 32 bit or 16 bit mixed precision. And the two kind of stayed in two different camps.
What you see now is a typical supercomputer would run one problem across a lot of nodes, and in AI workloads people are doing the same thing. MLPerf (DCK: an AI hardware performance benchmark) was just announced, with large numbers of nodes doing a single job. The workload between AI and HPC is actually very similar now. With our latest GPUs, the same GPU does traditional HPC double precision, does AI 32 bit precision, and does an accelerated AI mixed precision.
And traditional supercomputing centers are all doing AI now. They may have built a classic supercomputer, but they’re all running classic supercomputer tasks and AI on the same systems.
Same architecture for both. In the past, supercomputing used different networking than traditional AI. Now that’s all converged. That’s part of why we’re buying Mellanox. Right now, that backbone of supercomputing infrastructure, InfiniBand, is really critical to both sides. People thought of it as “just an esoteric HPC thing.” But no, it’s mainstream; it’s in the enterprise now, as the backbone for their AI systems.
Nvidia's DGX-2 supercomputer on display at GTC 2018
Is competition from all the alternative AI hardware, such as Google’s TPUs, FPGAs, other custom silicon designed by cloud providers and startups) a concern for Nvidia?
We always watch competition, but if you look at our competitors, they don’t benchmark against each other. They benchmark against us. Part of the reason that we’re so prolific in the industry is we’re everywhere. In the same Google cloud you’ve got Nvidia GPUs; in the same Amazon cloud, you’ve got Nvidia GPUs.
If your laptop had an Nvidia GPU, you could do training on that. Our GPUs just run everything. The software stack you could do deep learning training on your laptop with is the same software stack that runs on our top 22 supercomputers.
It’s a huge issue when all these startups and different people pick one benchmark: “We’re really good at ResNet 50.” If you only do ResNet 50, that’s a minor part of your overall AI workload, so having software flexibility and having programmability is a huge asset for us. And we built an ecosystem over the last decade for this.
That’s the biggest challenge I think to startups in this space: you can build a chip, but getting millions of developers to use your chip when it’s not available inside your laptop and in every cloud is tough. When you look at TPU (Google’s custom AI chip), our latest MLPerf results, we submitted in every category except one, where TPU only submitted in some workloads where they thought they were good.
It’s good to have competition, it makes you better, but with the technology we have, the ecosystem that we have, we’ve got a real advantage.
Traditional HPC architecture converging with AI means the traditional HPC vendors are now competing with DGX. Does that make your job harder?
I don’t see them as competition at all, because they all use Nvidia GPUs. If we sell a system to a customer, or HPE, Dell, or Cray sells a system to a customer, as long as the customer’s happy, we have no issue.
We make the same software we built to run on our own couple thousand DGX systems internally available through our NGC infrastructure (DCK: NGC is Nvidia’s online distribution hub for its GPU-optimized software), so all of our OEM customers can pull down those same containers, use that same software, because we just want everyone to have the best experience in GPU.
I don’t view any of those guys as competition. As a product-line owner, I share a lot with my OEM partners. We always build DGX first because we need to prove it works. And then we take those lessons learned and get them to our partners to shorten their development cycle.
I’ll have meetings with any of those OEMs, and if they’re looking at building a new system, I’ll tell them OK, over the last two months that I’ve been trying to build a new system, here’s what I ran into and here’s how you can avoid those same problems.
Is there any unique Nvidia IP in DGX that isn’t shared with the OEMs?
The unique IP is the incredible infrastructure we built inside Nvidia for our own research and development: all of our autonomous cars, all of our deep learning research, that’s all done on a few thousand DGX systems, so we learn from all of that and pass on those learnings to our customers. That same technology you can find in an HPE, a Dell, or a Cray system.
One of the common things we hear from customers is, ‘Hey Nvidia, I want to use the thing you use.’ Well, if you want to use the thing that we use every day, that’s a DGX system. If you’re an HPE shop and you prefer to use HPE systems because of their management infrastructure, that’s great. They built a great box, and the vendor that you’re dealing with is HPE at that point, not Nvidia.
But from a sales and market perspective, we’re happy as long as people are buying GPUs.
Google recently announced a new compression algorithm that enables AI workloads to run on smartphones. Is there a future where fewer GPUs are needed in the data center because phones can do all the AI computing?
The world is always going to need more computing. Yeah, phones are going to get better, but the world’s thirst for computing is ever-growing. If we put more computing in the phone, what does that mean? Richer services in the data center.
If you travel a lot, you’ve probably run into a United or an American Airlines voice-response system: it’s gotten a lot better in the last few years, because AI is improving voice response. As it gets better, you just expect more services on it. More services means exponentially more compute power. Moore’s Law is dead at this point, so I need GPUs to accomplish that task. So, the better features you put on the phone, the better business is for us. And I think that’s true of all consumer services.
Have you seen convincing use cases for machine learning at the mobile-network edge?
I think that’s coming online. We’re engaged with a lot of telcos at the edge, and whether you think about game streaming, whether you think about personal location services, telcos are always trying to push that closer to the customer, so that they don’t need the backhaul as much. I used to work for telco companies a decade ago or so, and that thirst for moving stuff to the edge is always there. We’re just now seeing some of the machine learning applications that’ll run at the edge. As 5G rolls out, you’re only going to see more of that stuff.
What kind of machine learning workloads are telcos testing or deploying at the edge?
It’s everything for user-specific services. If you’re in an area, the applications on your phone already know you’re in that area and can give you better recommendations or better processing. And then, as people start to consume more and more rich content, as bandwidth improves, more processing will move to the far edge.
While telcos are the ones pushing compute to the edge, are they also going to be the ones providing all the rich services you’re referring to?
Sometimes they’re building services, sometimes they’re buying services. It’s a mix, and I think that’s where the explosion in AI and ML apps is today. You’ve got tons of startups building specific services that telcos are just consuming at this point. They’re coming up with great ideas, and the telco distribution network is an ideal place to put those types of services. A lot of those services need a lot of compute power, so the GPUs at the edge I think are going to be a compelling thing going forward.
About the Author
You May Also Like