DCW' 23: Deploying Generative AI Without GPUs or SupercomputersDCW' 23: Deploying Generative AI Without GPUs or Supercomputers
Developers can use second-hand, CPU-based legacy hardware to do the compute.
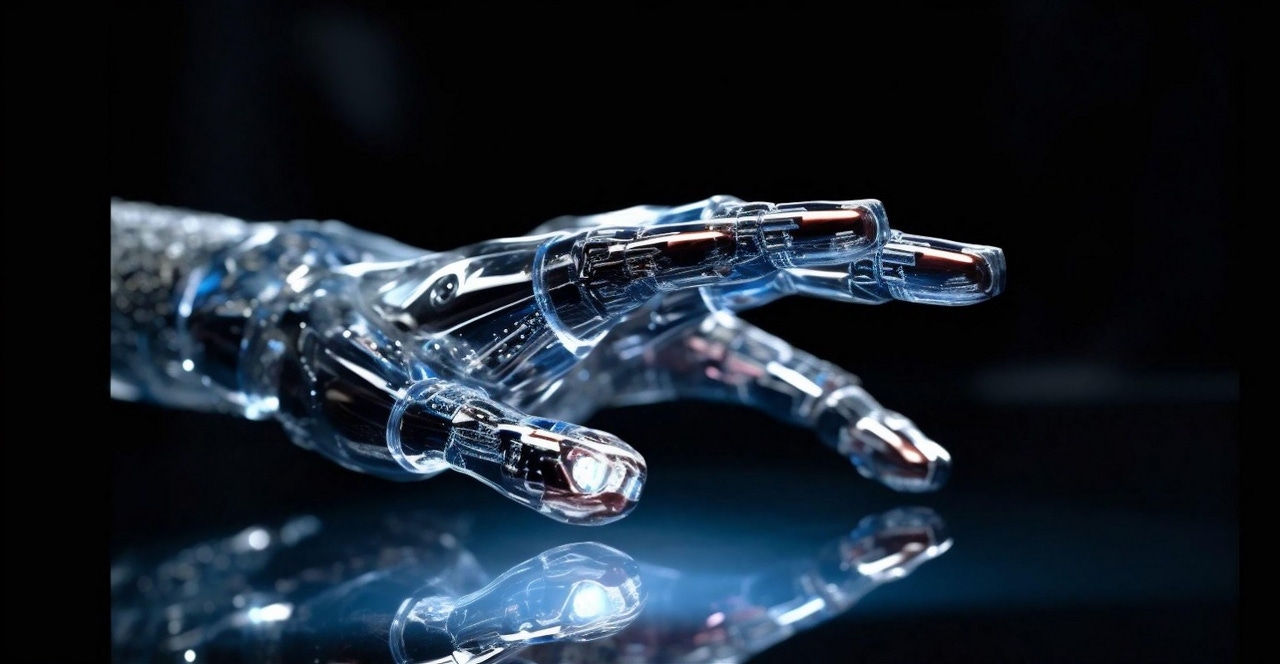
Technological innovations that generate a lot of hype typically have to face harsh reality at some point when practitioners begin deployment. For AI, especially generative AI, that reality is starting to sink in.
"Training (a large language model) is extremely costly," said Constantine Goltsev, partner at AI/ML solutions agency theMind, during a recent panel at Data Center World 2023 in Austin, Texas.
With ChatGPT's 175 billion parameters, he said, it meant OpenAI had to do 175 billion calculations of the input to produce results, using up gigawatts of power overall. With GPT-4, OpenAI used 12,000 to 15,000 Nvidia A100 chips — each costing $10,000 — on Azure and ran compute for months.
The good news is companies do not have to use gigantic language models because much smaller, open source ones can deliver results that are just as good as ChatGPT or even surpass it.
"You don't necessarily need the large language model on the industrial scale, like ChatGPT or GPT-4, to do a lot of useful stuff," Goltsev said. "You can take smaller academic models or open source models on the order of 6 billion parameters, 3 billion parameters, and then you can fine-tune them using exactly the same methods that are used to create ChatGPT. And the results are very decent."
For example, if a large law firm wants to build a semantic search engine that can go through a mound of legal case files comprising terabytes of data, it can go to AWS and get a few of its large instances, about eight A100 cards and a lot of memory and storage. The cost would be about $30 to $40 an hour.
Then, fine-tuning a mid-size GPT model with 64 billion parameters for a couple of weeks should cost the law firm about $30,000 to $50,000. "That will produce a pretty decent result for you to work with," Goltsev said. …
About the Author
You May Also Like