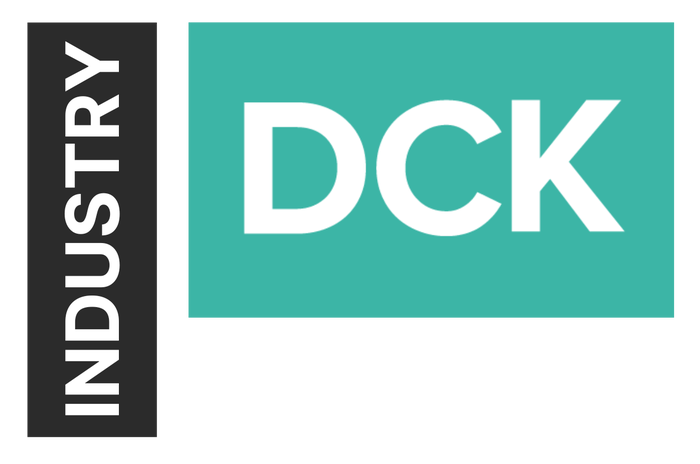
Insight and analysis on the data center space from industry thought leaders.
How an Operational Data Hub Becomes the Data Detective
With the amount of data created and stored growing exponentially, discovering the relationships that exist among your data requires more than a keen mind. It requires the right technology, too.
January 30, 2017
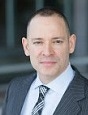
Ken Krupa is CTO at MarkLogic.
Data sleuths inclined to using logic and the science of deduction to ferret out patterns and establish links between clues take enjoyment in solving data problems. However, with the amount of data created and stored every day growing exponentially, discovering the relationships that exist among your data requires more than a keen mind. It requires the right technology, too.
The Covert Culprit: Data Silos
For many organizations, data lies in multiple, disconnected data silos resulting from earlier departmental initiatives. The inability to undertake complex data integration across silos means organizations are missing out on a full view of their data, which can bring revenue-generating insights and customers’ preferences, and avoiding the risk of fines due to difficulty complying with regulations.
Mergers and acquisitions can create even more of these silos and the reality of multiple copies of data being spread out across these disjointed systems presents the organization with serious data integrity issues. Compounding the complexity, from financial services firms to multi-national pharmaceutical companies – today’s businesses are under mounting pressure to comply with ever changing regulations, all the while looking over their shoulders for the next disruptive business model that will invariably come along to challenge their established positions.
Solve the Disparate Data Conundrum with an Operational Data Hub
The good news is that the right technology implementation – an operational data hub (ODH) – can help organizations solve their data integration challenges and extract more value from their data. An operational data hub is an active integrated data store/interchange that can hold a single, unified 360-degree view of all of your data. Up to 80 percent of today’s enterprise data is in unstructured, or multi-structured format such as office documents, PDFs, message exchange formats, mobile data, digital metadata as well as silos of varying RDBMS models. Because of the variance, it makes sense to build this operational data hub on a database built to handle all types of data. This is where an Enterprise NoSQL database fits the bill because it can ingest any type of data, and eliminate time-consuming data wrangling and ETL (extraction, transformation and loading) processes that can take years to implement and cost millions to maintain – all inherent weaknesses part and parcel of traditional relational databases.
To choose the right Enterprise NoSQL database, look for integrated search and semantic capabilities as well as full enterprise-grade ACID compliance. Semantics makes it easier to discover these inferred facts and relationships, integrating concepts and categories and providing context. When a database has ACID capability, even the largest datasets are processed consistently and reliably so none of the data is ever corrupted or lost. Importantly, due to the scalability and agility of NoSQL, the system can also be quickly adapted, extended and enhanced to meet changing business and regulatory requirements.
Here are real-world examples of operational data hubs solving complex data integration challenges:
The Case of Rampant Risk in the Investment Banking Industry
Investment banks need to ensure their trade data is high quality, accessible and searchable to mitigate risk and maintain compliance with regulatory imperatives, such as MiFID II, FRTB and Dodd-Frank.
A leading investment bank built an operational data hub using an Enterprise NoSQL database. This technology enabled a single view of derivatives trades and provides a full audit trail for auditors and regulators. It also replaced 20 RDBMS database instances with a single Enterprise NoSQL database cluster, making trade information retrievable as well as actionable in real-time. And not only has the database enhanced the investment bank’s compliance reporting, it has dramatically reduced its maintenance costs as the system is built on a commodity scale-out architecture. The result is not-only a lower cost per trade but an orders-of-magnitude faster time to delivery whenever business needs change. The bank can now develop and deploy new software – and therefore launch new products in response to the market – much more quickly.
Combining technical innovation with insights, another leading investment bank needed to maintain and demonstrate compliance with Dodd-Frank data analysis processes. Using Enterprise NoSQL’s bitemporal data management feature allowed the bank to minimize risk through “tech time travel” – time stamping and “rewinding” the state of their data to identify changes by looking at the data as it was over the course of time without having to reload data backups.
The Case of Ineffective Fraud Detection in the Insurance Industry
Fraud detection rates in the insurance industry remains frustratingly low. Most fraud is only noticed after the crime has been committed and it’s become too expensive to recoup the money lost.
Current rates of fraud detection that rely solely on human expertise are, at best, 10 percent of the total committed – and frequently lower. By using an operational data hub, insurers can take advantage of the combined power of big data, semantics and inference to detect previously unknown fraudulent behavior. What’s more, instead of a passive read-only interaction with the data, an operational data hub with semantics capability allows investigators to interact with the data in a more conversant read-write way, providing a two-way platform that combines the power and scale of computing with human intuition. With such an active and operational 360-degree view of the data, it becomes possible to evaluate a fraud claim within its context, provide additional inferred context as needed and perform comparisons to potentially similar claims in order to identify patterns.
Rules may also be set up and enhanced based on discovered patterns. When properly equipped to analyze data, assign a risk score to each claim, alert the right people in real-time and delay payment to settle all high scoring suspicious claims, insurers will soon be in a position to successfully detect fraud at a rate that saves billions of dollars each year.
All Signs Point to a Data Strategy Breakthrough
Regardless of format or source, the devil is in the details when it comes to an organization’s data and its ability to delve into those data details can become a crucial business differentiator. A flexible operational data hub allows businesses to take advantage of the power of big data, semantics and inference to gain crucial insights. When it comes to complex data integration and building an advantageous 360-view of your business, you don’t have to go it alone and neither does your data.
Opinions expressed in the article above do not necessarily reflect the opinions of Data Center Knowledge and Penton.
Industry Perspectives is a content channel at Data Center Knowledge highlighting thought leadership in the data center arena. See our guidelines and submission process for information on participating. View previously published Industry Perspectives in our Knowledge Library.
About the Author
You May Also Like