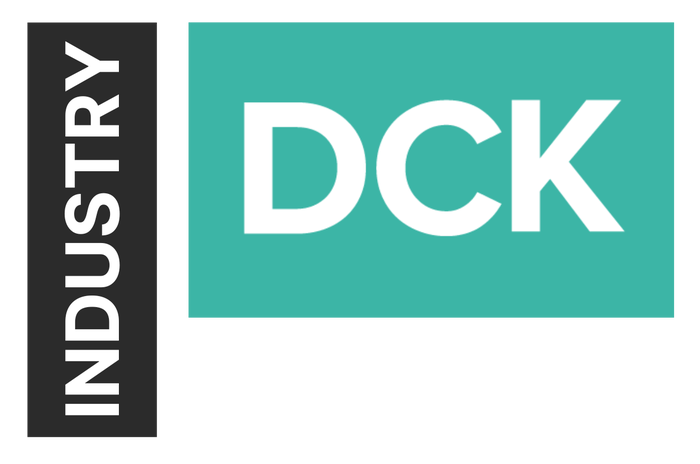
Insight and analysis on the data center space from industry thought leaders.
Cloud Apps Are Getting Smarter
Companies may still be reluctant to move to cloud apps because they don't want to give up critical predictive functionality that is baked into their core business processes, such as cross-sell, next best activity, or churn management, writes John Ball of KXEN. Lost revenue due to losing cross-sell capability would be staggering for a medium to large-scale contact center. But there are means to address this through predictive analytics.
September 17, 2013
John Ball is CEO of KXEN, which performs predictive analytics. He brings 20 years of experience in enterprise software, deep expertise in business intelligence and CRM applications, and a proven track record of success driving rapid growth at highly innovative companies.
john-ball-tn
JOHN BALLKXEN
Cloud apps are hot. There may have been questions 10 years ago if businesses were ready to put their mission-critical business processes outside of their four walls, but today the cloud is mainstream. Whether it’s CRM, ERP or HR, cloud apps are bringing huge value to companies of all sizes. Enough so that Gartner estimates that over 40 percent of CRM revenue was attributed to SaaS in 2012 and the market will increase to over 50 percent by 2016. Not too shabby.
I was recently at a Salesforce customer event in San Francisco, and Marc Benioff had his usual lineup of leading companies like GE, Omnicom, and some newcomers like Trunk Club all running their customer-facing interactions on salesforce.com. You simply can’t help but get caught up in all the excitement.
Barriers Remain
But amidst all this momentum, some companies are still reluctant to move key business processes to the cloud. The old excuses of security, scalability, latency, and uptime have been resolved for the most part. So why is there still hesitation? The main reason is these companies cannot give up critical predictive functionality that is baked into their core business processes, such as cross-sell, next best activity, or churn management. Lost revenue due to losing cross-sell capability would be staggering for a medium to large-scale contact center (often processing hundreds of thousands or millions of calls a month).
From what I can tell, it's the only remaining advantage that on-premise apps like Oracle, SAS, IBM Unica, SAP, and Pegasystems still have over their cloud peers.
Predictive Analytics Answers the Questions
Customer interaction processes such as cross-sell, churn management, and personalized recommendations are made smarter through predictive analytics. Predictive engines analyze the mountains of data collected from CRM systems, web, mobile traffic, and social media (called Big Data these days) and deliver answers to questions such as “which customer is likely to leave my service?” or “which product is the customer most likely to purchase?” The end result is that the interactions are more profitable for the company and more relevant for the customer.
So if predictive applications are so important, then what’s the hold up?
The reality is that delivering predictive applications isn’t easy. Most existing predictive applications have been custom developed involving teams of PhD data scientists and lots of IT heavy on-premise plumbing. Delivering predictive applications for cloud apps introduces fundamentally new design requirements. Specifically, there are three major challenges, which need to be addressed:
1. Automation: You simply can’t have thousands of data scientists sitting in the cloud handcrafting predictive models for every marketing offer, retention program, website recommendation, etc. Traditional predictive modeling is a manual, iterative process that takes days or weeks to produce a single model. In a multi-tenant cloud such as salesforce.com, there are over 150,000 customers, each with a different data and underlying data models, making any attempt at handcrafted predictive modeling for each customer conversation an impossible task.
2. The Physics Problem: Most companies have distributed data. The data resides in multiple cloud systems (e.g. CRM in salesforce.com, ERP in Netsuite, web traffic in Adobe) and often there are still on-premise data sources (e.g. billing data). The laws of physics still precludes pumping terabytes of data back and forth, so predictive engines need the ability to analyze and score in a distributed environment.
3. Packaged Applications: Cloud customers don’t typically know what data science is so they need packaged applications with simple UIs. These businesses need the application to embed the same best practices for cross sell, upsell, retention that have been proven in the on-premise world for cloud customers that don’t have the time, money or skills to build them themselves.
There’s no doubt that the introduction of predictive applications into cloud systems can provide tremendous value. Some of the most successful businesses have relied on similar techniques on-premise to run their business. But for these companies to fully adopt cloud applications, they need the confidence that cloud vendors can provide these same capabilities.
There are many start-ups now focusing on big data and predictive analytics. Some are targeting the traditional analytics market dominated by SAS and IBM with modern approaches, while others are focusing on new distributed infrastructures such as Hadoop. It’s time to take the best of the various new approaches and make cloud systems smarter.
Industry Perspectives is a content channel at Data Center Knowledge highlighting thought leadership in the data center arena. See our guidelines and submission process for information on participating. View previously published Industry Perspectives in our Knowledge Library.
About the Author
You May Also Like