Not Just for Google: ML-Assisted Data Center Cooling You Can Do Today
Cooling management software that uses machine learning algorithms has been around for years. Here’s how and where it’s been used.
November 9, 2018
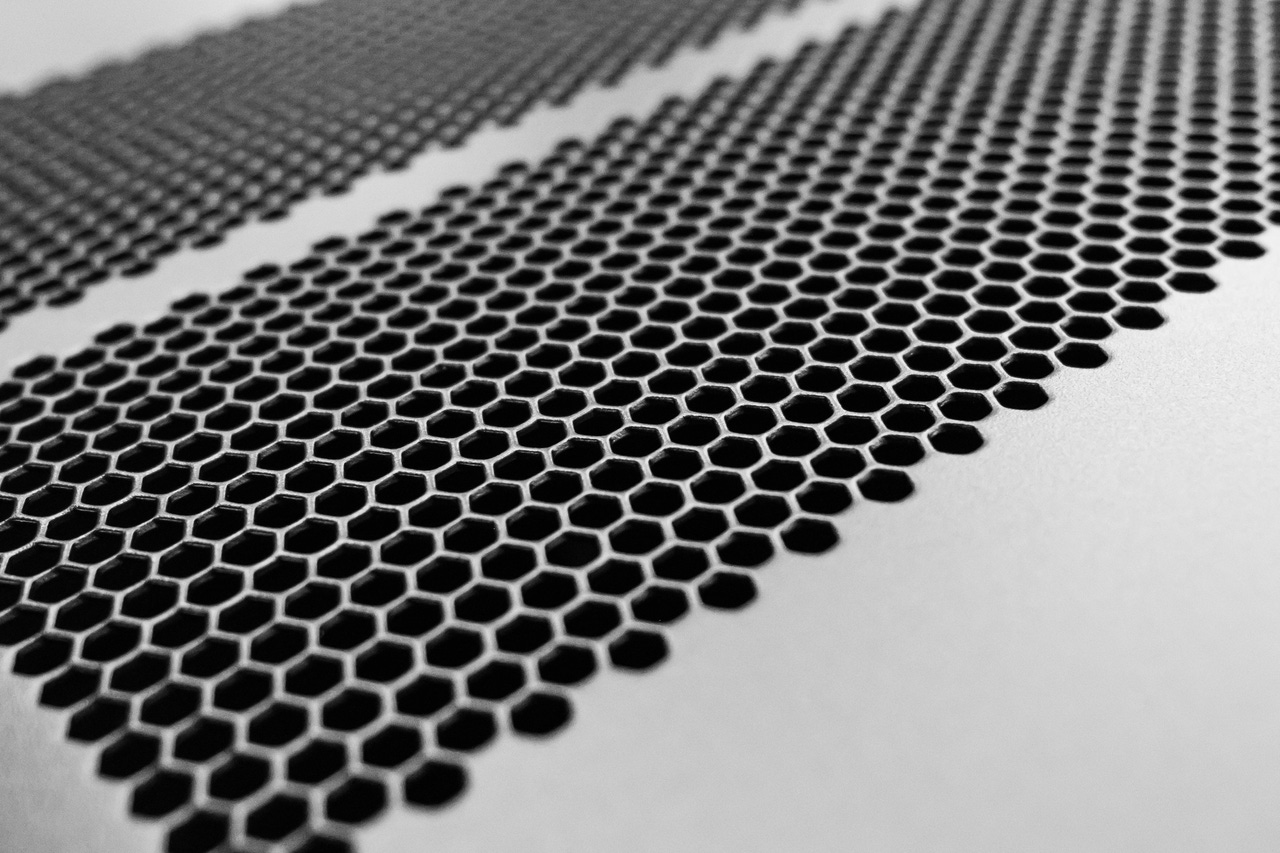
Many enterprises routinely over-cool their data centers with the goal of preventing failure. Not only is this blunt-force approach extremely inefficient, it doesn’t guarantee that none of the IT equipment will overheat.
“You encounter hot spots even in an over-cooled data center,” Rajat Gosh, CEO of AdeptDC, a startup whose software use machine learning to manage data center infrastructure, told Data Center Knowledge in an interview. One of the hardest problems to solve in data center cooling is pressure distribution, he said, and machine learning can be especially effective at solving it.
Earlier this year, Joe Kava, the man in charge of data centers for Alphabet’s Google, revealed to us that the company had been using machine learning algorithms to automatically tune its data center cooling systems, which enabled cooling energy savings of up to 30 percent. Google has considered turning the technology into a solution it can offer to other companies managing industrial facilities, and it may that sometime in the future, but you don’t need to wait.
It may not have been done in the exact same way before, but the concept of applying machine learning to data center cooling management isn’t new. A software company called Vigilent has been offering technology that does this for about a decade. It’s in use in more than 600 data centers, or some 19 million square feet of floor space combined, the company claims. And it has customer references to back up its claims. Verizon, for example, saves about 55 million kilowatt-hours of energy per year at 24 data centers, while NTT has reduced its cooling costs by 32 percent, according to Vigilent. The company’s software is built into data center systems by Hitachi Vantara, Siemens, and Schneider Electric (including Schneider’s EcoStruxure Data Center Management as a Service offering).
How Much Cooling Do You Really Need?
AdeptDC is after a similar opportunity, but, while both companies are using machine learning, they approach the problem in different ways. One big difference is how they gather the operational data that trains their ML models. AdeptDC relies on the temperature readings from server CPUs, while Vigilent uses sensors placed across the data center floor to understand not just the temperature profile, but also the specific impact of individual cooling units and their interactions. It uses this data to build what it calls an “influence map.” (We explained AdeptDC’s approach in depth in another recent post.)
A misconfigured or failed cooling unit, for example, would be shown on the map as having little or no influence, Cliff Federspiel, Vigilent president and CTO, explained to us. The results are often counterintuitive, which is an area where ML is especially effective, since it doesn’t rely on intuition like humans do. A data center technician may hear a cooling unit run, see it vibrate, feel a cool air stream coming out of it, and conclude that it’s performing as expected. But the influence map may show that it’s making no contribution to cooling the IT gear inside the facility.
“You can also figure out which cooling units are super important,” so you can pay special attention to them, Federspiel said. “Because if it fails, it will have a bigger effect on the temperature of the floor than if another were to fail.” In other cases the data may show that there’s enough cooling redundancy for most of the room, while one local spot only relies on a single unit. That spot may house some critical equipment that would fail if that one unit fails, wreaking havoc for the organization.
Misdirected Air
The influence map can also uncover more fundamental airflow issues, such as badly placed perforated tiles. Such basic issues are more widespread than one might assume. “Almost every site we go into that has a raised floor or ducted airflow, the air delivery is really not very effective,” Federspiel said. “The visualizations we have are very effective at helping get manual air delivery back to where it needs to be.”
The rise of free cooling (which is now mandated for new US data centers) brings its own problems, especially if summer temperatures require a combination of free and mechanical cooling, which is difficult to configure correctly and prone to failure. “More than half the time customers are not getting their money’s worth for their free cooling,” Federspiel said.
Vigilent also uses machine learning to make daily operations more efficient by running “what-if” scenarios and turning cooling units on and off briefly to discover optimization opportunities. “A lot of these buildings are designed to be highly redundant and have extra capacity, but they’re not designed with the instrumentation and telemetry to know how much extra capacity there is,” he said. In a use case similar to Google’s, the software can change temperature setpoints, slow fans down, or turn cooling units off entirely to save energy, delivering only the amount of cooling the current IT workload needs – and it retrains periodically to stay up to date.
If the software indicates significant overcapacity, data center operators can add more servers and racks without adding more cooling or repurpose existing cooling equipment. It’s also possible to use Vigilent to move workloads around the data center to take full advantage of the available cooling capacity, Federspiel said.
Applicable Across the Board
Today, cooling optimization is the most mature and widely adopted use of machine learning in data center management, Rhonda Ascierto, a research VP at Uptime Institute, told us. “Software that automatically adjusts the setpoint of a cooling unit or slows down fans or turns off fans” could be in as much as 15 percent to 25 percent of the data center market, she estimated.
“It’s applicable to all types of cooling systems, including free cooling, whether that’s direct or indirect adiabatic cooling; even though you may not be dealing with mechanical systems, it can still be used for louvre control,” she pointed out. But managing mechanical cooling is where machine learning shines brightest, so the rise of direct outside-air cooling and adoption of more efficient cooling systems could tamper the demand. “You get the biggest bang for the buck with more inefficient cooling systems. There are a lot of middle-aged data centers out there; there are a lot of older, mechanically cooled data centers out there.”
Equipment vendors are promoting adoption of machine learning in their own ways, which include adding more instrumentation to their equipment. “This is part of a broader strategy by some equipment suppliers to enable more machine learning-driven out-of-the-box dynamic cooling optimization,” Ascierto said. “Prefab modular data centers, including micro data centers, increasingly will integrate machine learning cooling out of the box.”
Vertiv’s iCOM Autotuning, for example, uses machine learning to control settings across all cooling center components, such as compressors, fans, and condensers, as a single system. The company claims its approach makes the whole cooling system up to 15 percent more efficient and improves equipment service life by reducing wear.
Predictive Maintenance and Risk Analysis
Another big driver for adoption of machine learning in data center management is predictive maintenance. “You can automate maintenance,” Ascierto said. “If the bot says this cooling unit will have an issue in the next two months, we can have someone come fix it in six weeks’ time.”
In her estimate, risk analysis could eventually outstrip efficiency as the main driver for adoption. “You can understand your risk profile from a cooling perspective at any given point in time, and how that changes as the reliability of all equipment drifts over time, as the configuration of the equipment changes, as you add more IT equipment to the room,” she said. “Although efficiency has been the main focus, over time, risk analysis will be a bigger driver.”
About the Author
You May Also Like