Data Lakes Evolve: Divisive Architecture Fuels New Era of AI Analytics
The once-simple data lake continues to evolve to drive enterprise analytics. That matters more today as AI knocks on the corporate door.
September 12, 2024
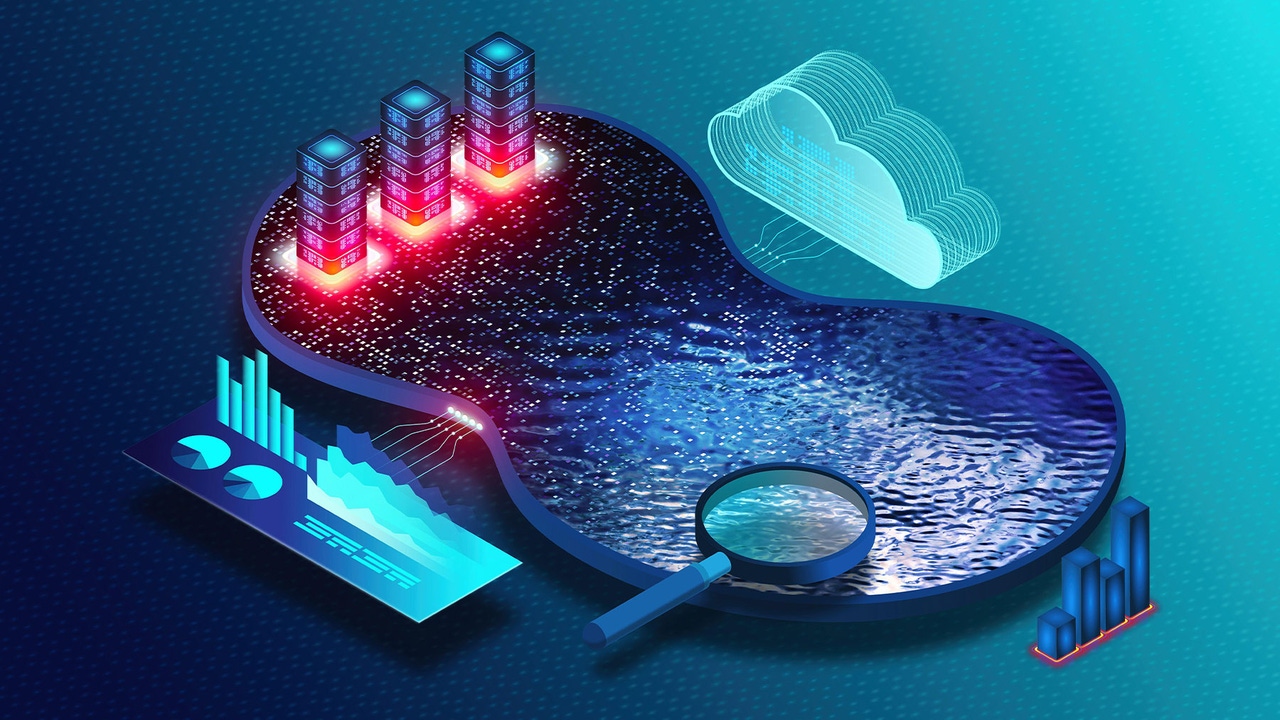
When the idea arose in the early 2010s, the data lake looked to some people like the right architecture at the right time. The data lake was an unstructured data repository leveraging new low-cost cloud object storage formats like Amazon’s S3. It could hold large volumes of data then coming off the web.
To others, however, the data lake was a ‘marketecture’ that was easy to deride. Folks on this side called it the ‘data swamp.’ Many in this camp favored the long-established – but not inexpensive – relational data warehouse.
Despite the skepticism, the data lake has evolved and matured, making it a critical component of today’s AI and analytics landscape.
With generative AI placing renewed focus on data architecture, we take a closer look at how data lakes have transformed and the role they now play in fueling advanced AI analytics.
The Need for Data Lakes
The benefits of implementing a data lake were manifold for young companies chasing data-driven insight in e-commerce and related fields.
Amazon, Google, Yahoo, Netflix, Facebook, and others built their own data tooling. These were often based on Apache Hadoop and Spark-based distributed engines. The new systems handled data types that were less structured than the incumbent relational data types residing in the analytical data warehouses of the day.
For the era’s system engineers, this architecture showed some benefits. ‘Swamp’ or ‘lake’, it would come to underlay pioneer applications for search, anomaly detection, price optimization, customer analytics, recommendation engines, and more.
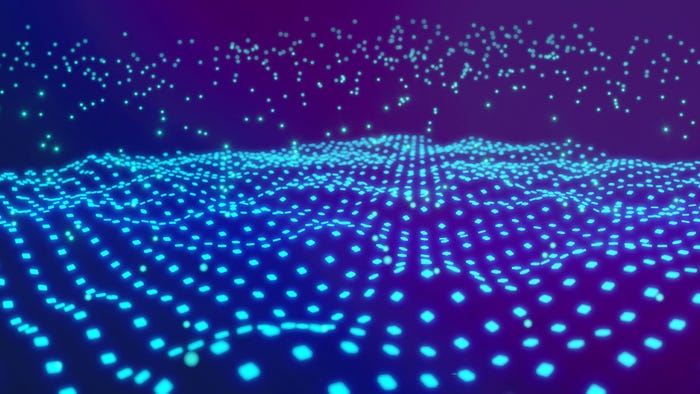
Drop in the ocean: Data lakes hold vast, untapped potential – storing massive amounts of data today to drive tomorrow’s insights and AI advancements.
This more flexible data handling was a paramount need of the growing web giants. What the author of Distributed Analytics, Thomas Dinsmore, called a “tsunami” of text, images, audio, video, and other data was simply unsuited to processing by relational databases and data warehouses. Another drawback: Data warehousing costs rose in step as each batch of data was loaded on.
Loved or not, data lakes continue to fill with data today. In data handling, data engineers can ‘store now’ and decide what to do with the data later. But the basic data lake architecture has been extended with more advanced data discovery and management capabilities.
This evolution was spearheaded by home-built solutions as well as those from stellar start-ups like Databricks and Snowflake, but many more are in the fray. Their varied architectures are under the microscope today as data center planners look toward new AI endeavors.
Data Lake Evolution: From Lakes to Lakehouses
Players in the data lake contest include Amazon Lake Formation, Cloudera Open Data Lakehouse, Dell Data Lakehouse, Dremio Lakehouse Platform, Google BigLake, IBM watsonx.data, Microsoft Azure Data Lake Storage, Oracle Cloud Infrastructure, Scality Ring, and Starburst Galaxy, among others.
As shown in that litany, the trend is to call offerings ‘data lakehouses,’ instead of data lakes. The name suggests something more akin to traditional data warehouses designed to handle structured data. And, yes, this represents another strained analogy that, like the data lake before it, came in for some scrutiny.
Naming is an art in data markets. Today, systems that address the data lake’s initial shortcomings are designated as integrated data platforms, hybrid data management solutions, and so on. But odd naming conventions should not obscure important advances in functionality.
In the updated analytics platforms today, different data processing components are connected in assembly-line style. Advances for the new data factory may center around:
New table formats: Built on top of cloud object storage, Delta Lake and Iceberg, for example, provide ACID transaction support for Apache Spark, Hadoop, and other data processing systems. An oft-associated Parquet format can help optimize data compression.
Metadata catalogs: Facilities like Snowflake Data Catalog and Databricks Unify Catalog are just some of the tools that perform data discovery and track data lineage. The latter trait is essential in assuring data quality for analytics.
Querying engines: These provide a common SQL interface to high-performance querying of data stored in a wide variety of types and locations. PrestoDB, Trinio, and Apache Spark are among examples.
These improvements collectively describe today’s effort to make data analytics more organized, efficient, and easier to control.
They are accompanied by a noticeable swing toward the use of ‘ingest now and transform later’ methods. This is a flip on the data warehouse’s familiar data staging sequence of Extract Transform Load (ETL). Now, the recipe may instead be Extract Load Transform (ELT).
By any name, it’s a defining moment for advanced data architectures. They arrived just in time for new shiny generative AI efforts. But their evolution from junk-draw closet to better-defined container developed slowly.
Data Lake Security and Governance Concerns
“Data lakes led to the spectacular failure of big data. You couldn’t find anything when they first came out,” Sanjeev Mohan, principal at the SanjMo tech consultancy, told Data Center Knowledge. There was no governance or security, he said.
What was needed were guardrails, Mohan explained. That meant safeguarding data from unauthorized access and respecting governance standards such as GDPR. It meant applying metadata techniques to identify data.
“The main need is security. That calls for fine-grained access control – not just throwing files into a data lake,” he said, adding that better data lake approaches can now address this issue. Now, different personas in an organization are reflected in different permissions settings.
This type of control was not standard with early data lakes, which were primarily “append-only” systems that were difficult to update.
New table formats changed this. Table formats like Delta Lake, Iceberg, and Hudi have emerged in recent years, introducing significant improvements in data update support.
For his part, Sanjeev Mohan said standardization and wide availability of tools like Iceberg give end-users more leverage when selecting systems. That leads to cost savings and greater technical control.
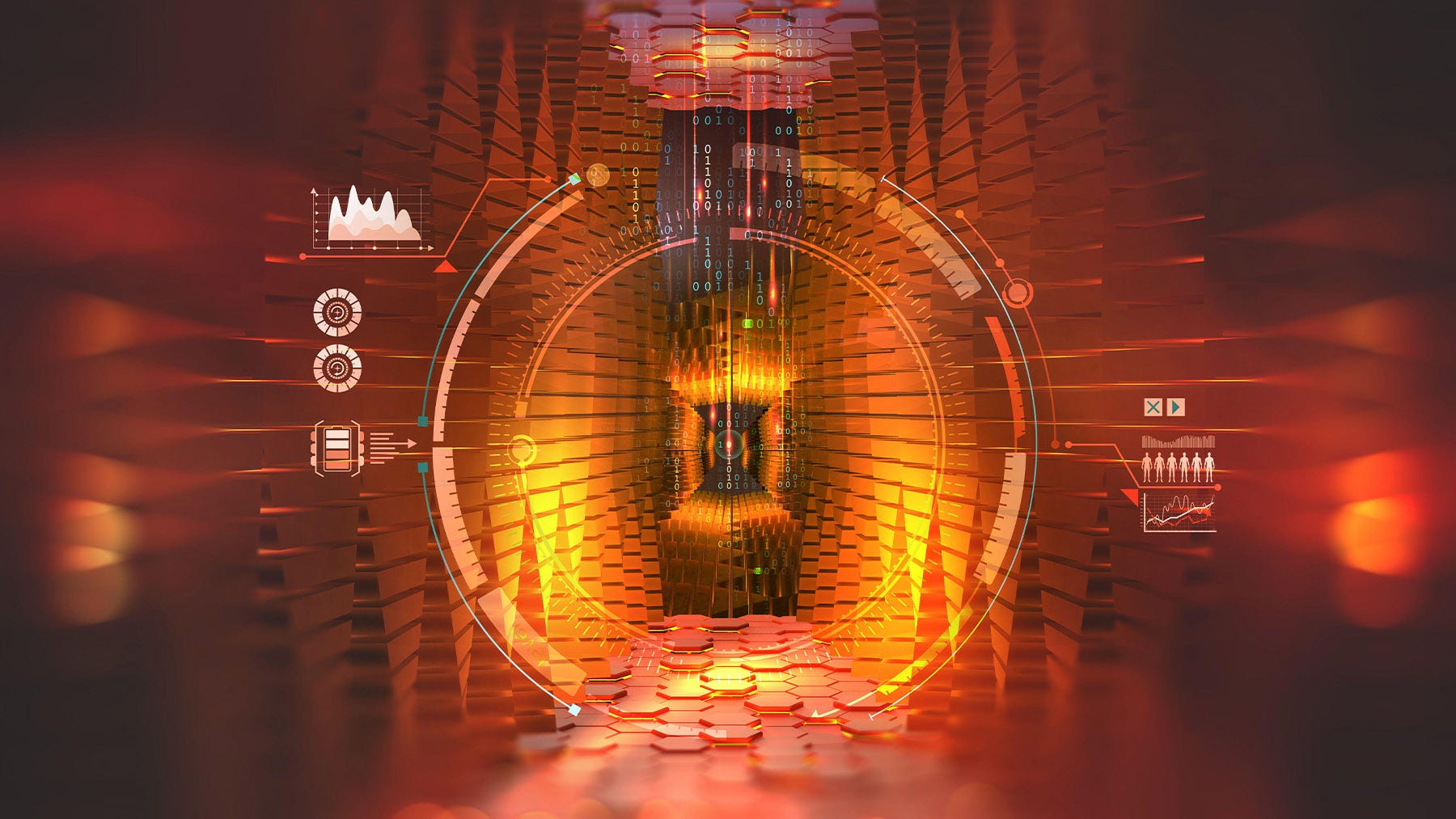
Fueling the future: Data lakes are powering advanced AI analytics by handling massive volumes of unstructured data.
Data Lakes for Generative AI
Generative AI tops many enterprises’ to-do lists today, and data lakes and data lakehouses are intimately connected to this phenomenon. Generative AI models are keen to run on high-volume data. At the same time, the cost of computation can skyrocket.
As experts from leading tech companies weigh in, the growing connection between AI and data management reveals key opportunities and hurdles ahead:
‘Gen AI Will Transform Data Management’
So says Ganapathy “G2” Krishnamoorthy, vice president of data lakes and analytics at AWS, the originator of S3 object storage and a host of cloud data tooling.
Data warehouses, data lakes, and data lakehouses will help improve Gen AI, Krishnamoorthy said, but it is also a two-way street.
Generative AI is nurturing advances that could greatly enhance the data handling process itself. This includes data preparation, building BI dashboards, and creating ETL pipelines, he said.
“With generative AI, there are some unique opportunities to tackle the fuzzy side of data management – things like data cleaning,” Krishnamoorthy said. “That was always a human activity, and automating that was challenging. Now we can apply [generative AI] technology to get fairly high accuracy. You can actually use natural-language-based interactions to do parts of your job, making you substantially more productive.”
Krishnamoorthy said a growing effort will find enterprises connecting work across multiple data lakes and focusing on more automated operations to enhance data discoverability.
‘AI Data Lakes Will Lead to More Elastic Data Centers’
That’s according to Dipto Chakravarty, chief product officer, Cloudera, a Hadoop pioneer that continues to provide new data-oriented tooling.
AI is challenging the existing rules of the game, he said. That means data lake tooling that can scale down as well as scale up. It means support of flexible computation at the data centers and in the cloud.
“On certain days of certain months, data teams want to move things on-prem. Other times, they want to move it back to the cloud. But as you move all these data workloads back and forth, there is a tax,” Chakravarty said.
At a time when CFOs are mindful of AI’s “tax” – that, is, its effect on expenditures – the data center will be a testing ground. IT leaders will focus on bringing compute to the data with truly elastic scalability.
‘Customization of the AI Foundation Model Output Is Key’
That’s how you give it the language of your business, according to Edward Calvesbert, vice president of product marketing for Watsonx Platform at IBM – the company that arguably spurred today’s AI resurgence with its Watson Cognitive Computing effort in the mid-2010s.
“You customize AI with your data. It’s going to effectively represent your enterprise in the way that you want from a use case and from a quality perspective,” he said.
Calvesbert indicated Watsonx data serves as the central repository for data within the Watsonx ecosystem. It now underpins the customization of AI models, which, he said, can co-locate within an enterprise's IT environment.
The customization effort should be accompanied by data governance for the new age of AI. “Governance is what provides lifecycle management and monitoring guardrails to ensure adherence to your own corporate policies, as well as any regulatory policies,” he said.
‘More On-Premises Processing Is in the Offing’
That is according to Justin Borgman, chairman and CEO of Starburst, which has parlayed early work on a Trino SQL query engine into a full-fledged data lakehouse offering that can pull data from beyond the lakehouse.
He said well-curated data lakes and lakehouses are essential for supporting AI workloads, including those related to generative AI. He said we will see a surge of interest in hybrid data architectures, driven partly by the rise of AI and machine learning.
“This momentum around AI is going to bring more data back to the on-prem world or hybrid world. Enterprises are not going to want to send all their data and AI models to the cloud, because it costs a lot to get it off there,” he said.
Borgman points to the use of query and compute engines that are essentially decoupled from storage as a dominating trend – one that will work within the diverse data infrastructures that people already have in place, and across multiple data lakes. This is often called “moving the compute to the data.”
Is More Data Always Better?
AI workloads that are based on unsorted, inadequate, or invalid data is a growing problem. But as data lake evolution suggests, it’s a known problem that can be addressed with data management.
Clearly, access to a large amount of data is not helpful if it cannot be understood, said Merv Adrian, independent analyst at IT Market Strategy.
“More data is always better if you can use it. But it doesn’t do you any good if you can’t,” he said.
Adrian positioned software like Iceberg and Delta Lake as providing a descriptive layer on top of vast data that will help with AI and machine learning styles of analytics. Organizations that have invested in these types of technology will see advantages when moving to this brave new world.
But the real AI development benefits come from the skilling teams gain from experience with these tools, Adrian said.
“Data lakes, data warehouses, and their data lakehouse off-shoot made it possible for businesses to use more types and more volume of data. That’s helpful for generative AI models, which improve when trained on large, diverse data sets.”
Today, in one form or another, the data lake abides. Mohan perhaps puts it best when he said: “Data lakes have not gone away. Long live data lakes!”
About the Author
You May Also Like