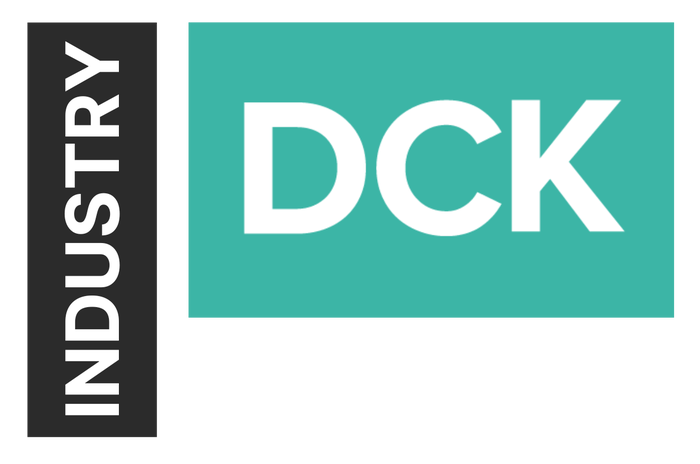
Insight and analysis on the data center space from industry thought leaders.
Building a Successful Data Lake: An Information Strategy FoundationBuilding a Successful Data Lake: An Information Strategy Foundation
Data lakes have had their ups and downs, but they’re here to stay — and have the power to unlock data’s true value.
September 12, 2018
Michael Hiskey is Head of Strategy at Semarchy.
As the Strata Data Conference begins this week in New York, it’s interesting to see how the big data proponents have all been able to rally around the data lake as a concept, with a side of Artificial Intelligence and Machine Learning to make it interesting.
Everyone is a Data Scientist
On the surface, it’s easy to see why data scientist has been considered “the best job in America,” three years in a row. The salary is stellar, the positions are endless, and the work is on the forefront of innovation.
To really understand the occupation, though, one must peek under the hood of any organization and see the data lake, the infrastructure for storing, accessing, and retrieving large volumes of data. In one word, the data lake makes data science possible.
So it’s been strange to watch as in recent years data lakes have been maligned as evil: big, generic, unwieldy, and always on the precipice of a swamp. All of these things can be true, but they can also be easily avoided with intelligent technologies.
Data lakes may have a slim margin for error — mismanage them for a moment and they self-corrupt — but that only reflects their relevance. In today’s world, a data lake is the foundation of information management — and, when built successfully, it can empower all end-users, even nontechnical ones, to use data and unlock its value.
How the Data Lake Formed
The first step is to build a successful data lake is to understand why data lakes are here to stay. Relational databases, which were created in the early ’70s, ensured that all data could be linked together. Using SQL, they allowed for easy look-ups of vast amounts of information and dominated the enterprise market for years.
It all changed after the the dotcom crash, in the Web 2.0 era. Armed with internet business wisdom and emerging technologies like Hadoop and NoSQL, organizations began digitizing. Information exploded with the big data movement in 2012, affecting everything from management practices to national elections.
Suddenly, business weren’t just collecting data from customers, they were producing data during operations. And products weren’t just creating data, products were data… and data itself became a product. It quickly dawned on organizations that the reams of information had to be worth a lot. But how could you know that as it all came streaming in?
The solution was the data lake. Put another way, the data lake is the conceptual retention of all raw data, without regard to how it will be used later. Don’t store the data just because it’s possible — store it because you know it will be valuable, once the data scientist unlocks the value.
The technical concept behind this is called “schema on read,” which juxtaposes with a “schema on write” concept. Put simply, either the data is put into a meaningful format upon “writing” to storage, or it is put there with no formatting, and making sense of it is done upon “reading” it later.
The Data Hub Brings Excellence to Every Layer
Like its namesake, the data lake is not a static object but a moving piece of nature. Just as a natural lake can become contaminated with chemical runoff and turn to toxic sludge, an unmaintained data lake risks turning into a data swamp.
The data hub controls for all of that. Built atop a data lake, it makes data available throughout the organization, from big data experts running business intelligence to nontechnical users who running operations and supporting customers. This brings relational data concepts back in, and marries the various conventional back end systems with the data lake.
By uniting data governance, master data management, data quality, and workflows, the data hub allows users to interact with current business systems, and control the access and auditability of that data. For instance, the data hub will allow a customer service rep to match and merge customer information with a single keystroke, ensuring seamless interoperability of customer data.
How the Data Lake Powers Innovation
What makes the data hub intelligent is the extra layers of Artificial Intelligence (AI) and Machine Learning (ML), innovative, almost futuristic technologies that bring logic and clarity to reams of information.
AI and ML are still created by people that treat all data as human data — that is to say, with care and respect — they’re just designed to interpret more information than a human could ever imagine, and then serve it up to end-users in real time, with an eye toward business goals.
This nexus of automation, technology layers, and databases is arguably civilization’s “Next Big Thing.” But to make them work in concert, companies must first ensure that the raw, infinite bits of information are in place. And that starts with the data lake and the data hub. If they succeed, everything else can succeed.
Opinions expressed in the article above do not necessarily reflect the opinions of Data Center Knowledge and Informa.
Industry Perspectives is a content channel at Data Center Knowledge highlighting thought leadership in the data center arena. See our guidelines and submission process for information on participating.
About the Author
You May Also Like