AI’s Impact on Data Center E-Waste and How to Mitigate the ProblemAI’s Impact on Data Center E-Waste and How to Mitigate the Problem
Despite the transformative potential of AI, its rise may exacerbate data center e-waste. Discover strategies to mitigate this growing environmental concern.
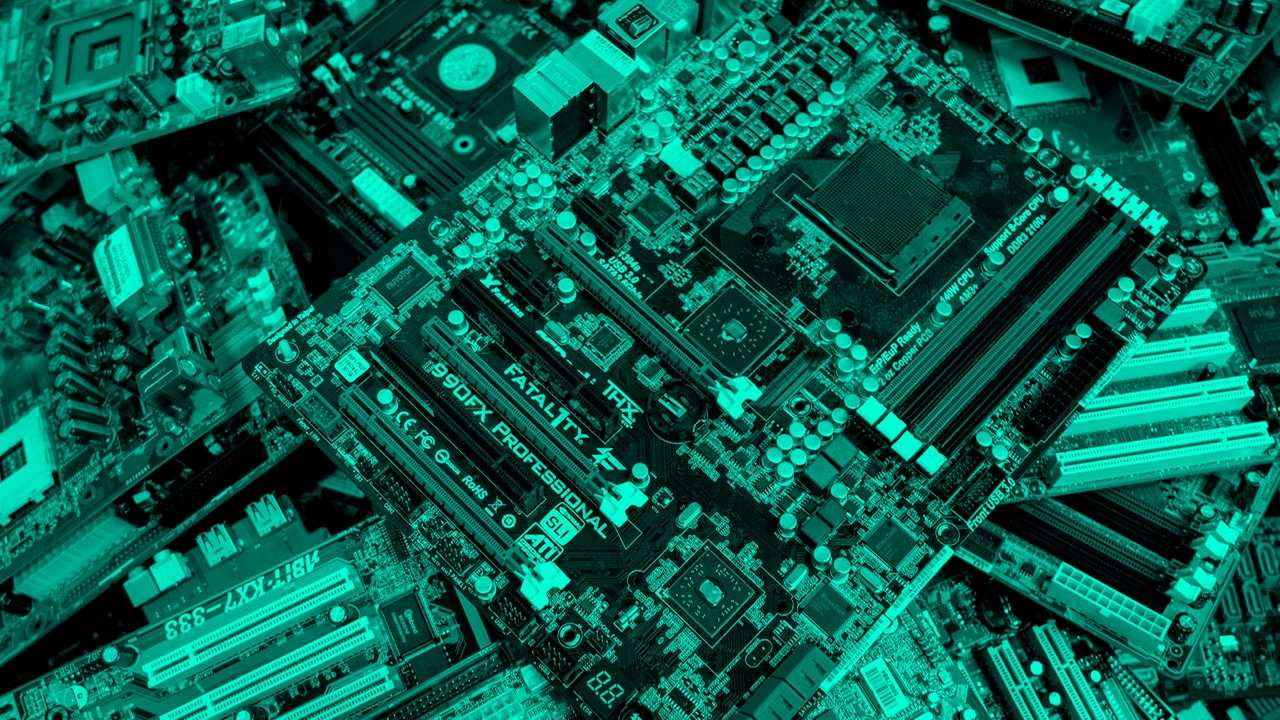
Electronic waste (e-waste) has long been a challenge for data center operators concerned about environmental sustainability and social responsibility. However, the ongoing boom surrounding AI could make the data center e-waste problem even worse.
That’s why now is the time for data center operators, as well as businesses that deploy AI workloads inside data centers, to start thinking about e-waste management strategies. By getting ahead of the issue, they can reduce the amount of AI infrastructure that results in e-waste.
Data Center E-Waste: The Basics
E-waste is any type of electronic product that is no longer in use and could potentially harm the environment. The equipment that data centers house – such as servers, network switches, and power supply units – can contain chemicals like lead and mercury. This means the equipment has the potential to become e-waste when it’s no longer in use.
E-waste is bad from an environmental sustainability perspective because dangerous compounds inside data center equipment can leach into the natural environment, potentially harming plants, animals, and humans. It can also negatively impact people in developing nations, which often become the final destination for discarded IT equipment.
Will AI Make E-Waste Worse?
As with many tech sectors, data centers have contributed to e-waste for decades. But this challenge could grow, as more and more businesses seek to take advantage of AI – especially generative AI.
The reason why is that generative AI applications and services must undergo a process called training, which involves parsing vast quantities of data to recognize patterns. Training typically takes place using servers equipped with Graphical Processing Units, or GPUs. GPUs are much faster for training than traditional CPUs because GPUs have a higher parallel computing capacity, which means they can process more data at the same time.
In most cases, AI training is a temporary or one-off process. Once an AI model has completed its training, it doesn't need to train again, unless its developers want to “teach” it new information. This means that training generative AI models is likely to result in the deployment of GPU-enabled servers for which there is not sustained demand.
After the training ends – in other words, after companies get AI models up and running – there will be less need for that hardware because there aren't many use cases for GPUs inside a data center beyond AI training, and most organizations won’t need to retrain on a frequent basis.
From an e-waste perspective, this has the potential to result in a number of GPUs – or entire GPU-enabled servers – with decidedly short lifetimes. They’ll still function but may become obsolete due to lack of demand.
A similar story has already played out in the cryptocurrency miningv realm – where GPUs and other specialized hardware are also important because they're often used for mining operations. Because equipment manufactured for cryptocurrency mining serves virtually no other useful purposes, much of it has become e-waste.
Mitigating Data Center E-Waste Caused by AI
The good news is that there are ways to avoid a massive uptick in data center e-waste caused by AI training.
One key step is for businesses to share AI training servers. Rather than purchasing their own GPU-equipped servers for training, companies can opt for GPU-as-a-Service offerings, which essentially let them rent GPUs. When they’re done training, the GPUs can then be used by another business that has a model to train. That's much more sustainable – not to mention more cost-effective – than owning GPU-enabled servers that don't require continuous use.
Read more of the latest data center sustainability news
Opting to use pre-trained models instead of building models from scratch is another way to help mitigate the e-waste risk of AI. A growing number of models are available from open source projects that have already been trained, eliminating the need for specialized data center infrastructure of any type.
Companies should also, of course, make sure they properly recycle or dispose of AI servers when they no longer need them. But ideally, they’ll minimize the number of servers they deploy in the first place that have the potential to become AI e-waste in short order.
Read more about:
Green ITAbout the Author
You May Also Like