A Smart Assistant for Data Center Cooling?A Smart Assistant for Data Center Cooling?
Startup AdeptDC applies machine learning to data centers’ biggest source of inefficiency: overprovisioning.
January 29, 2018
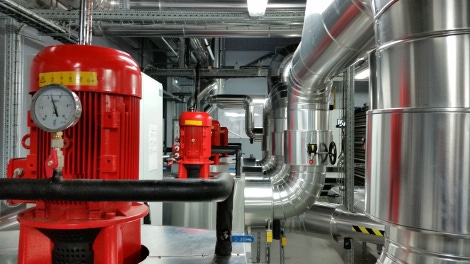
Does your data center actually need all the cooling capacity it has installed? If it’s like most data centers, it does not. That both cooling and power capacity in most data centers are significantly over-provisioned is not a secret.
Upsite Technologies figures are the most dramatic, with cooling capacities routinely four times higher than necessary:
On average, for most data centers, 75% of the cooling is not needed; that means if you spend $100 on cooling $75 of it is wasted. Gartner estimated 40% of cooling is wasted. Google has said with AI that they can save about 35% of cooling costs. Our initial testing shows that depending on the weather patterns, the outside temperature and the humidity of the outside air, and depending on the type of cooling, that you can save 25-40%.
Since most companies with data centers don’t have the kind of budget and expertise Google has to build new, efficient data centers from the ground up, a startup called AdeptDC wants to offer an off-the-shelf smart data center cooling system that’s entirely software based, using diagnostic information like CPU temperature measurements that avoid the time, cost, and complexity of fitting extra sensors to data center systems. And it works with any hardware and any type of cooling, the company claims. Gather that information for a full duty cycle, preferably over a month, and the machine learning-based thermal management system will suggest the changes to make to the cooling set-point in real-time – like a smart thermostat for the data center.
AdeptDC is based in Santa Clara, California, and lists pilot deployments with UPS, Georgia Tech, and Arrow Electronics.
“The biggest problem is that there’s no one to one correspondence between cooling and heat removal,” AdeptDC's CEO, Rajat Ghosh, told Data Center Knowledge. “You change the HVAC set-point, but that doesn’t guarantee the same change.” That’s because of the complexity of airflow and thermal issues like heat latency, he explained as well as the way IT workloads can change more quickly than cooling systems can respond, making it hard to predict the environment. “IT infrastructure processes change very fast but air cools slowly.”
You won’t get similar responses even from thermal systems in two identical data centers, he said. “Airflow is typically turbulent and non-deterministic. The air flow you get for a given set-point one day – there’s no guarantee you’ll get the same airflow the next day.” Because data center managers don’t really know how the system will behave under changing conditions, data center designers build in many layers of redundancy to make sure it’s ready for anything.
A screenshot from AdeptDC's tool
The cooling system is there for risk management. Looking at it as such, AdeptDC uses machine learning to improve the way operators manage risk and paint a clearer picture of what’s happening in the environment, aiming ultimately to help them facilities avoid overprovisioning.
It does that by working out what cooling is required based on the sources of heat in the data center infrastructure. “CPU temperature is the best possible indicator of the cooling you need to remove the waste heat that’s generated,” Ghosh said. The system can also read GPU temperature if your workloads use GPU compute. (Many of the world’s machine-learning workloads require dense clusters of GPUs for training, pushing proliferation of this type of processor in more and more data centers.)
Data center admins aren’t usually experts in thermal design and airflow management, and the combination of thermal complexity and the large volume of data make this a good problem for machine learning. “That’s where our AI solution comes in; we’re processing data in real-time and providing a very granular and low-latency set-point recommendation for the cooling systems to implement,” Ghosh said. “It’s like a digital thermal assistant.”
AdeptDC could help data center admins tackle smaller problems – like giving better visibility for a specific aisle that’s proving problematic – but it’s designed to work across the whole data center. The tool can also help you scale cooling capacity on-demand. “If the workloads, layout, or equipment of your data center changes, it’s easy to adapt cooling to the changing environment,” Ghosh told us.
Smart cooling could be particularly useful in edge data centers that may be in more demanding environments, in remote locations where you don’t have admin staff and want automated facility management, or where you just don’t have enough power available to run more cooling than you need. Using software rather than sensors simplifies installation in remote locations and means you don’t have to disclose those locations. “Many edge data centers are concerned about physical security,” Ghosh suggested.
A screenshot from AdeptDC's tool
With cooling being such a large proportion of the data center power budget, businesses need to understand what they’re spending that money on. Organizations trying to introduce usage-based pricing and chargeback models could use AdeptDC to understand which workloads contribute to cooling costs and allocate that to business budgets. Even without getting that specific, you can get a much better understanding of the efficiency of your data center than just looking at PUE.
Ghosh says PUE is myopic: “You're only looking at power and cooling; you’re missing a big part, which is the applications. Look at how much you’ve put in, how much operational gain you’re getting out of that capital investment, and then it becomes a business metric like return on investment.”
About the Author
You May Also Like