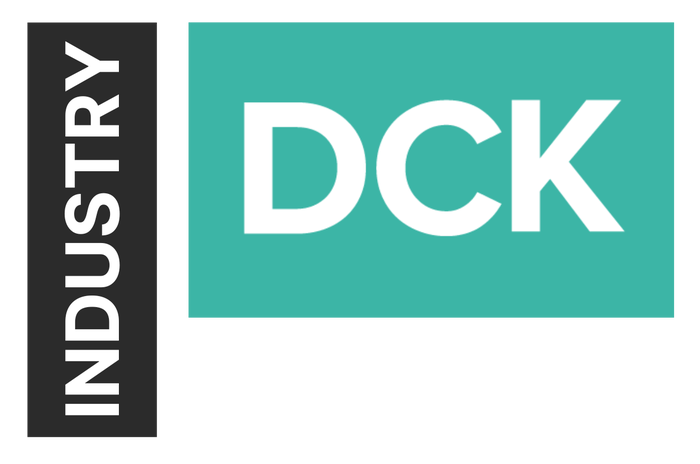
Insight and analysis on the data center space from industry thought leaders.
The AI/ML Revolution Is Upon Us, but Networking Pros Have Been Ready for It
AI/ML workloads exhibit similar characteristics and behaviors to HPC and HPD, meaning networking professionals can apply their existing knowledge base to ensure AI/ML runs as it should.
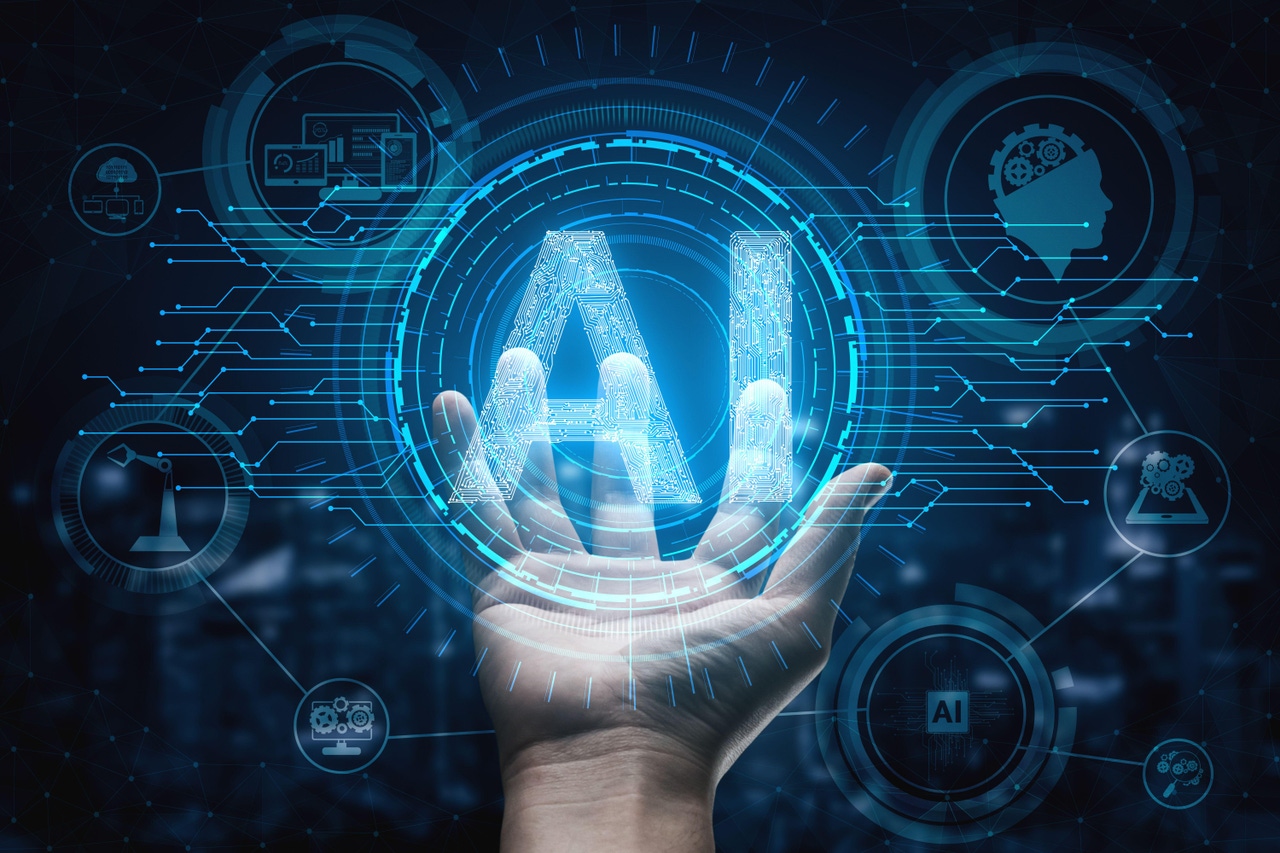
These days, it’s hard to avoid the topic of artificial intelligence (AI) and machine learning (ML). It’s everywhere. Even mainstream news is covering it regularly, mostly with curiosity of its vast potential for both limitless innovation and as a force disrupting the old ways of doing things.
What’s interesting about this trend is that the concept of AI/ML itself is not new. As a technology, it’s been around since 1956 when advanced computing researchers at Dartmouth University first coined the term “AI.” AI/ML has gone through a number of feast-or-famine cycles of investment and disinterest over the last seven decades. This newest cycle, however, looks to have legs and is likely to make headway, which will have implications for both application developers and underlying infrastructure providers.
But as powerful as AI/ML has become, supporting it as a workload is not necessarily new for network infrastructure operators. Many other workloads over the years, including voice, video, storage, high-performance computing (HPC), and high-performance databases (HPD) have helped harden IP and Ethernet networks to improve reliability, lower latency, guarantee lossless transmit, and increase performance. AI/ML as a workload on the network exhibits similar characteristics and behaviors to HPC and HPD, meaning networking providers and operators can apply their existing knowledge base to ensure AI/ML runs as it should.
There are also industry standard extensions that permit lossless transmission in the form of converged, enhanced Ethernet (also known as “lossless Ethernet”) that is now widely available to provide high throughput and low latency while avoiding traffic drops in situations where congestion occurs. This is certainly a sea change from the humble origin story of Ethernet as a best effort technology that became the de facto networking protocol for consumers and enterprises alike due of the global ecosystem of innovators and vendors who rallied behind it.
What Networking Pros Need To Know About AI/ML
This is not to say that there’s nothing unique or challenging about supporting AI/ML as a workload. Deploying and managing AI/ML workloads are not a set it-and-forget-it proposition because AI/ML at scale has two distinct deployment stages each with their own set of requirements.
The first stage is deep learning where humans train AI/ML computers to process vast amounts of data via learning models and frameworks. The goal is for machines to eventually be able recognize complex patterns in pictures, text, sounds, and other data to generate insights, recommendations, or even more advanced products. This is generally a compute-intensive stage requiring huge processing power and high-performance networking in terms of speed and capacity. It’s more than timely that both 400 and 800 Gigabit Ethernet technology are now widely available in the latest generation networking platforms.
The second stage is inference, which is the application part of AI/ML. ChatGPT is a prime example that involves humans querying machines in natural language, and these platforms responding in kind. The machines must be able to respond quickly for use cases such as language or picture recognition to ensure optimum user experience. Reducing network latency and reducing or eliminating network congestion are key requirements at this stage. Technologies such as the latest version of Remote Direct Memory Access over Converged Ethernet (RoCEv2) will prove their mettle as a way to achieve a lossless network that takes advantage of high throughput and low latency devices to transfer information between computers at the memory-to-memory level, without burdening the compute processors.”
One Network To Manage Them All
Irrespective of the stage, it is inevitable that AI/ML clusters will grow in size and complexity. This will require the networking industry to evolve its approach to how it builds scalable and sustainable networks optimized for AI/ML.
Today, IT organizations typically run separate networks based on the workload or the processor technology. It is no secret that AI/ML runs best on computers equipped with graphics processing units (GPUs), which are highly specialized processors attuned for latency-sensitive applications. The networking protocol of choice for GPUs has often been InfiniBand, a back-end technology designed to enable high-speed server-to-server communications. Conversely, IT has been using Ethernet as a front-end technology to support a variety of other workloads powered by ubiquitous central processing units (CPUs).
The growing trend for IT is to simplify operations wherever possible, including reducing the number of workload-specific networks. The overall goal is to reduce complexity, lower operational costs, and enable common best practices. The wide availability of converged/lossless Ethernet technology is making this a reality. IT organizations can leverage their existing Ethernet networks to support smaller AI/ML clusters (built with relatively few GPUs) by simply adding some new leaf switches and making minor configuration changes.
However, to support large-scale AI/ML clusters, there must be a measure of future proofing to make Ethernet the networking protocol of choice. This will include 400/800G networks (or even higher) delivered via ultra-high bandwidth networking silicon that can scale to 51.2 terabits per chip today. In addition, networking providers are “baking in special sauce” to further improve lossless behavior in Ethernet (e.g., the development of technologies such as distributed scheduled fabrics (DSF)).
Why Not Just “Go to the Cloud”?
Of course, one option for companies is simply to outsource their entire AI/ML compute, storage, and network infrastructure to one or more public cloud providers who offer this as a service. Public cloud providers have made considerable investments in GPUs, which makes it possible for their customers to ramp up quickly as GPU availability in the market is all too finite. However, as with any public vs. hybrid cloud debate, each customer must consider different factors to determine their best path forward when it comes to building their AI/ML clusters. This includes costs, data sovereignty, available skillsets, time to value, and other factors.
How To Get Started
Like Rome, AI/ML wasn’t built in a day. As mentioned previously, the road to AI/ML mass adoption has been a long one with many fits and starts along the way. Companies should keep this in mind as they embark on their own AI/ML journeys. A few best practices to help them may include:
One, start small and with what they already have, as existing networking hardware and software may be enough to support AI/ML as a workload in the initial phases with a few upgrades and adjustments.
Two, ask a lot of questions and weigh their options. Many different networking vendors will offer a wide range of solutions tailored to AI/ML. There are many ways to approach the AI/ML challenge so it’s important for companies to work strategically with vendors on sensible and practical solutions that are optimized for their needs.
Three, when ready, make the investment to future proof their network for AI/ML and other workloads yet to emerge. Networking is evolving more than ever and it’s a great time for companies to invest in modernizing their networking infrastructure for whatever the future holds.
About the Author
You May Also Like