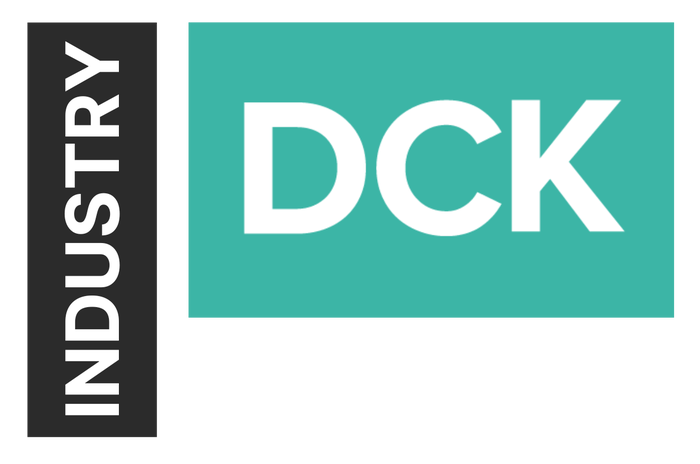
Insight and analysis on the data center space from industry thought leaders.
Data: Currency for the Fourth Industrial Revolution
Today, intelligent machines and services have ushered in the fourth industrial revolution – and infrastructure is scrambling to keep pace.
November 14, 2017
Brian Schwarz is VP of Product Management at Pure Storage.
Throughout human history, we have seen societies transformed from rural to industrial, from industrial to mass production, and from mass production to digital. Today, intelligent machines and services have ushered in the fourth industrial revolution – and infrastructure is scrambling to keep pace. Intelligent machines and services are expected to give rise to new industries, make many existing ones obsolete, and significantly transform society.
In healthcare, Mayo Clinic neuro-radiologists use artificial intelligence to identify genetic markers in MRI scans, which allows doctors to avoid retrieving tumor tissue through intrusive surgical procedures. Amazon has leveraged the same technologies that power self-driving cars, like sensor fusion and AI, to concept Amazon Go, a grocery store without check-out lines. Even traditionally rural industries, like farming, have put AI to use – LettuceBot harvests 10 percent of the lettuce crop in the U.S. using AI, which studies each plant in real-time to optimize yields.
The rise of AI has been fueled by the rise of three key technologies – deep learning (DL), Graphics Processing Unit processors (GPUs), and the ability to store and process very large datasets at high speed. All are major breakthroughs that have completely upended traditional approaches to innovation.
Deep learning is a new computing model that uses massively parallel neural networks inspired by the human brain. Instead of experts handcrafting software, a deep learning model writes its own software by learning from a huge pool of examples. A GPU is a modern processor with thousands of cores, best-suited to run algorithms that loosely represent the parallel nature of the human brain.
Which leads us to the third piece – big data. Within the last two years, the amount of computing power required to run bleeding-edge deep learning algorithms has jumped 15x. Compute delivered by GPUs has jumped 10x. But while the volume of unstructured data has exploded, legacy infrastructure that has not fundamentally changed in decades simply cannot unlock its full value. Deep learning and GPUs are massively parallel, but legacy technologies were not designed for these workloads – they were designed in an era with an entirely different set of expectations around speed, capacity and density requirements.
Today, we’re in the midst of a dramatic shift in the nature of data and the types of tools available to analyze it. As a result, entire business models have begun – and will continue – to evolve alongside. While Hadoop was the only widely available analytics tool a decade ago, data scientists have many tools at their disposal today. Apache Spark is a real-time, streaming framework that’s simpler and more powerful than Hadoop. Kafka is a real-time messaging tool for any file sizes, small or large. Hive offers a SQL-like interface that results in random, not sequential, accesses. The list goes on, and the result is an impending transformative operational impact across nearly every industry.
If data is the new currency for the fourth industrial revolution, the system that delivers the data should not be based on decades-old building blocks that will inevitably slow machine learning performance. Imagine a thirsty marathon runner trying to hydrate post-race through a wafer-thin straw – essentially, this is what happens to organizational data run on yesterday’s platform.
Ultimately, much of the actionable insights remain locked in the data. To truly take advantage of the fourth industrial revolution, there is a great need for innovation – a new data platform that is reimagined from the ground-up for the modern era of intelligent analytics.
As data pushes beyond the limits for which legacy technologies were designed a modern approach demands an architecture that is real-time, dynamic and massively parallel. A dynamic data hub on which any workload can grow on-demand, in compute or in capacity, delivering the highest performance for any unstructured data must have these six key qualities:
Tuned for Everything: unstructured data can have any size, form, or access pattern and the data hub must deliver uncompromised performance for any data.
Real-Time: many modern applications, like Spark, are designed for streaming data.
All-Flash: dramatically faster than spinning, mechanical disks, with random, low-latency access.
Parallel: from software to hardware, data hub should be massively parallel end-to-end, without any serial bottlenecks.
Elastic: today’s tools are built cloud-first and assume infrastructure is agile and elastic like cloud.
Simple: researchers and engineers want to focus on data, not infrastructure management. This means easy administration, but also rock-solid, proven reliability, resilience and availability.
A data platform must rise up to meet the dynamic needs of the new era. It should be simple, stable and elastic to power your way through the marathon ahead. As AI and deep analytics move beyond theory and academia into real-world application, be sure you’re ready to take advantage.
Opinions expressed in the article above do not necessarily reflect the opinions of Data Center Knowledge and Informa.
Industry Perspectives is a content channel at Data Center Knowledge highlighting thought leadership in the data center arena. See our guidelines and submission process for information on participating. View previously published Industry Perspectives in our Knowledge Library.
About the Author
You May Also Like