Nvidia Data Center Chief: On-Prem GPU Deployments for AI RisingNvidia Data Center Chief: On-Prem GPU Deployments for AI Rising
Hyperscale cloud platforms are a big GPU market, but big enterprises often have reasons to keep AI infrastructure in-house.
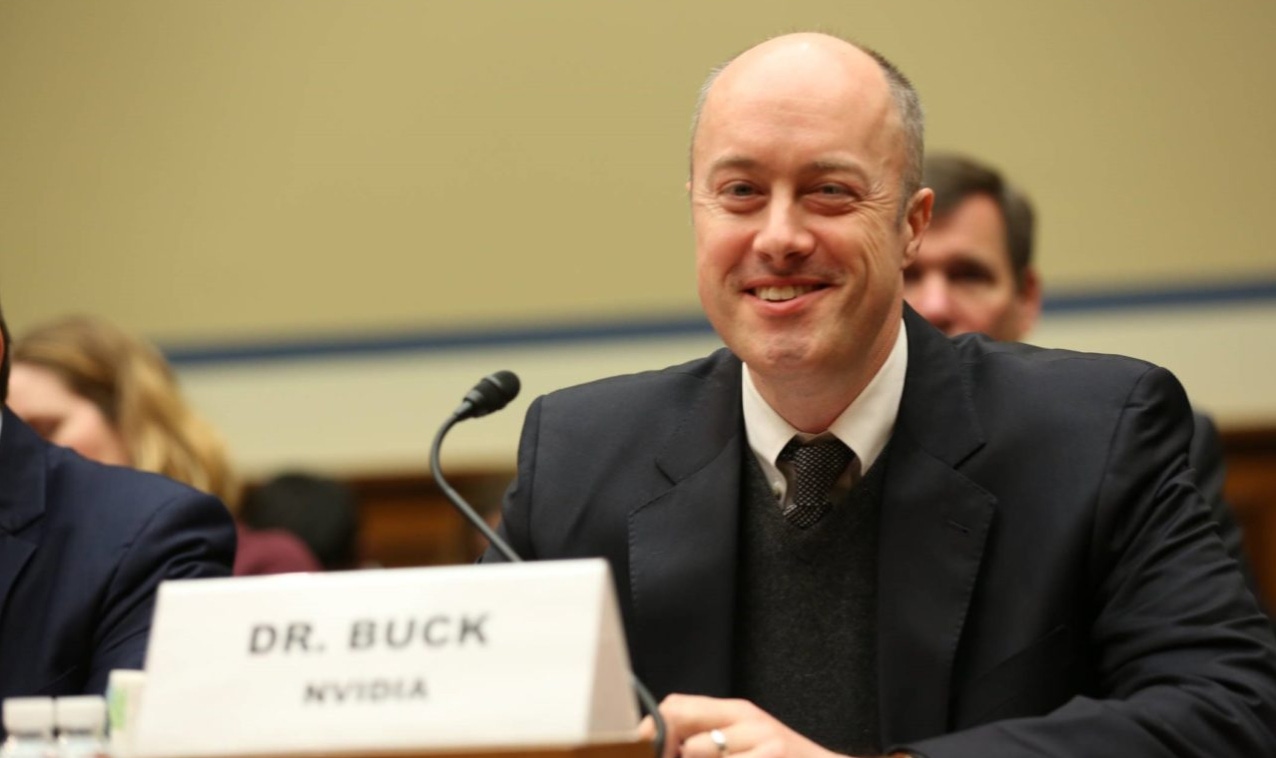
When he’s not fielding reporters’ questions about Nvida’s latest AI chips at a conference or educating a congressional committee in Washington about the importance of investing in AI research, Ian Buck is in the AI-infrastructure trenches, pushing the limits of chip design to make data centers capable of handling ever more powerful AI applications.
Riding the wave of AI enthusiasm, Nvidia’s stock reached a record high last week ahead of the release of its first-quarter earnings announcement. Expectations that its data center business, which Buck leads as VP and general manager, would once again report a stellar quarter drove the surge. And the group didn’t disappoint, reporting 71 percent more revenue than last year. (The stock dropped following the release, as the market reacted to the company revealing for the first time that a bigger-than-expected portion of its total revenue came from the unpredictable cryptocurrency mining space.)
Data Center Knowledge recently caught up with Buck to ask him about the latest trends in deployment of computing infrastructure that underpins AI applications in data centers. Here’s the full interview, edited for clarity and brevity:
DCK: Have you seen a pick-up in on-premises GPU deployments for machine learning applications by enterprises?
Ian Buck: Certainly. We’re seeing folks like SAP, Salesforce, eBay start to train neural networks and deploy their neural networks on GPUs for inferencing in on-prem environments. It’s a choice for these companies. Do they want to rent those GPUs in the cloud or use their on-prem servers.
What’s usually the advantage of using them on-prem?
Some IT departments are quite capable of managing their own infrastructure. They can optimize it and customize it specifically to their own needs, not just what the CSPs offer. They also have concerns about data privacy, especially financial information, user information. It’s more comforting to have data on their own premises, on their own servers that they control.
That said, CSPs care a lot about data privacy and are doing their best to prove that they have the most secure infrastructure. And they actually do a very good job at that.
So, it’s a matter of preference and choice, and sometimes economics as well. Certainly, it’s a rent-versus-buy decision, and the economics is different in every case.
Have you seen certain business verticals deploying GPUs on-prem more than others?
Certainly, we’re seeing more adoption in self-driving vehicles. They need to have large systems for simulating and training these neural networks. That’s definitely a large growth area.
There’s a lot of interest in healthcare. Healthcare is an area where there is a massive amount of medical data. The impetus is there to help turn that data into cures, into solutions to fight diseases. There’s a ton of activity going on now to figure out how can neural networks and deep learning be used to solve some of these diseases to understand the basics of cancer, to do better diagnosis, to provide better imaging.
Other than that, people are looking at traditional data analytics. We have companies like SAP are investing heavily in providing new kinds of services like brand insight, where they can actually recognize brands in video streams and sporting events, so they can provide better services to their customers – using deep neural networks for recommender systems, for better advertising, for better click rates. These new kinds of enterprise services are starting to show up.
Which verticals are the most advanced in using deep learning on GPU infrastructure?
The large hyperscalers obviously have a lot of data and were first to market with deep neural networks.
SAP is doing amazing work. I’d definitely take a look at what they’re doing. Another company that’s doing really well is actually GE. They’re starting to innovate on doing predictive maintenance. Being able to use AI that can ingest all of the data that’s coming off sensors on gas turbines used to generate energy. By being able to basically look at all that data and process it with AI, they’re actually able to service the gas turbine before it actually fails.
Do you see them deploying AI computing infrastructure at the edge?
There’s more opportunities there for sure of being able to monitor the entire fleet. They’re actually doing some interesting work with drones to observe and look for failures on sites like oil rigs to identify problems early and take action before something more expensive or more catastrophic could occur.
Do you expect most enterprise machine learning workloads to eventually run in hyperscale clouds?
No, I think there will always be a mix. I’m not sure what the actual mix will end up being. Certainly, the hyperscale cloud service providers are very interested in providing that service. There’s a certain convenience to using those services for some companies. Other companies deem it strategically important that they own the asset, and that’s one of their differentiations. They can tune and design their data center architecture, the infrastructure, the InfiniBand switches, and the network topologies to build perhaps more supercomputers than straight-up clusters.
Do you think the basic reasons for keeping this type of infrastructure on-prem are the same as the reasons for keeping other types of workloads on-prem?
The on-prem decision is multi-faceted. Some companies have top-tier IT and know how to build these large kinds of supercomputers. Some of them have specific optimizations in their architectures that they can build. It’s just not a product that the CSPs are interested in offering right now. Then there’s data privacy of course, and then there’s the economics, if you can invest in a system and run it for a very long time. It’s a buy-versus-rent economics.
How do the new Volta GPUs change data center power density?
The Volta GPU, the first issue, V100, it was a successor to Tesla P100, the previous Pascal-based GPU. We kept the power the same, yet we delivered over 3x more deep-learning training in the same configuration, at the same power, same size, same mechanicals. The infrastructure that could deploy a previous server like DGX-1, with Pascal, could also deploy Volta. [DGX-1 was the first supercomputer Nvidia built as its own product.]
Jensen Huang, founder and CEO, Nvidia, unveiling the DGX-2 supercomputer at GTC Summit 2018
That’s very important to us. We understand that designing servers is really hard. We do it, obviously, ourselves now and understand that complexity. We do make changes – like NVlink for example. We do that purposefully, where we can deliver 8x, just in six months, of AI performance. [Nvidia unveiled the much more powerful DGX-2 supercomputer in March, just six months after it launched the predecessor.] We’re not afraid of breaking the rules, but we do so thoughtfully and with large benefits in delivered performance and efficiency.
What has been the impact of Google’s TPUs on Nvidia’s GPU business?
We haven’t seen much of an impact. It actually, if anything, has shown how acceleration is a really important part of neural networks. Google themselves offer cloud GPUs.
In general, we’re getting a lot more interest and continue to grow. This space is huge. AI and deep learning is transforming how we think about software, how we design our hardware, how we program our computers, literally software writing software. The many people figuring out new ways, and techniques, and new architectures that make sense – like Google, and others as well – that’s fine, and I think it’s great. There’s many companies that have talked about building AI ASICS and such things. We are going to do what we do, which is continue to build an amazing platform for developing next-generation AI, for training those neural networks and deploying them for inferencing.
About the Author
You May Also Like